연관성 규칙 탐사에서의 대칭적 순수 신뢰도의 제안
Proposition of Symmetrically Pure Confidence in Association Rule Discovery
- 한국자료분석학회
- Journal of The Korean Data Analysis Society (JKDAS)
- Vol.13 No.2
-
2011.04879 - 889 (11 pages)
- 3
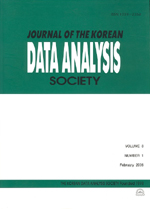
연관성 규칙은 데이터 마이닝 분야에서 가장 많이 활용되고 있는 데이터 마이닝 기법으로, 대용량 데이터베이스에 내재되어 있는 각 항목들 간의 관련성을 수치화함으로써 항목집합들간의 관련성을 나타내는 기법이다. 의미 있는 연관성 규칙을 탐사하기 위한 가장 기본적인 흥미도 측도에는 지지도, 신뢰도, 향상도 등이 있으며, 이들을 이용하여 연관성 규칙을 생성하게 된다. 이 때 사용되는 신뢰도는 비대칭적 측도인 동시에 계산된 값만을 가지고는 양의 연관성을 가지는지 음의 연관성을 가지는지를 알 수 없어서 음의 연관성을 가지는 연관성 규칙을 의미 있는 양의 관계를 가지는 규칙으로 선택하게 되는 오류를 범할 수 있다. 이를 해결하기 위해 본 논문에서는 대칭적 순수 신뢰도를 제안한 후, 예제를 통하여 기존의 신뢰도 및 대칭 신뢰도와 비교함으로써 대칭적 순수 신뢰도의 유용성을 알아보았다. 그 결과, 신뢰도와 대칭적 신뢰도는 모두 양의 값을 가지므로 항목집합들 간에 양의 연관성이 있는지 아니면 음의 연관성이 있는지를 알 수 없는 반면에, 대칭적 순수 신뢰도는 그 값이 취하는 부호에 의해 연관성 규칙의 방향을 알 수 있었다.
The exploration of association rules is one of the well-studied problems in data mining and its task is to discovery certain association relationships among a set of data items in a huge database. There are some primary quality measures for association rule (support, confidence, symmetric confidence, lift, etc). We generate some meaningful association rules using these measures. Support and lift are symmetric measures but confidence and symmetric confidence are asymmetric measures. If we use confidence and symmetric confidence for association rule generation, we cannot know whether the association is positive or negative by their values, and so we may reach the wrong conclusion.In this paper we propose a symmetrically pure confidence and then compare the confidence, symmetric confidence, and symmetrically pure confidence using some simulation data. As the result, we could not distinguish the direction of association rule by confidence and symmetric confidence, but we knew whether the association is positive or negative by symmetrically pure confidence.
1. 서론
2. 대칭적 순수 신뢰도
3. 예제를 통한 유용성 고찰
4. 결론
참고문헌
(0)
(0)