절사와 가중치를 이용한 향상된 배깅 알고리즘에 대한 연구
A Study on Advanced Bagging Algorithm Using Trimming and Weighting
- 한국자료분석학회
- Journal of The Korean Data Analysis Society (JKDAS)
- Vol.13 No.2
-
2011.04869 - 878 (10 pages)
- 5
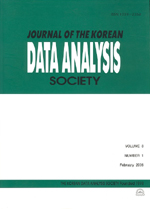
앙상블 기법을 이용하여 예측 알고리즘을 사용하는 가장 큰 이유는 변동성이 작으며 예측력이 뛰어난 알고리즘을 구축하기 위함이다. 그러나 대표적인 배깅 알고리즘들은 여전히 자료의 변동에 따라 예측의 정확도가 떨어지고 변동성이 커 다양한 형태의 자료에 대한 분류를 수행하는데 한계가 있다. 본 논문에서는 단일 분류방법론들을 결합하는 새로운 방법을 제안함으로써 예측의 정확성을 높이고 변동성을 줄이는 향상된 배깅 방법에 대한 연구를 하고자 한다. 기존의 배깅 알고리즘과 본 논문에서 제안한 방법을 실제자료에 적용시켜 향상된 정도를 파악함으로써 배깅 알고리즘이 보다 개선되었는지 여부를 비교 연구한다. 연구 결과 가중평균만을 이용한 배깅 방법은 기존 배깅 방법 보다는 예측력이 좋아졌지만 다른 수정된 배깅 방법론에 비하여 뚜렷한 향상정도를 보이지 못했다. 반면에 가중된 절사 배깅 방법은 모든 분류자에서 상대 향상도가 유의하게 양의 값을 가지는 것을 나타났는데, 기존 배깅 방법론의 예측력이 안정적인 기본 분류자를 사용했을 때 떨어진다는 단점을 보완할 수 있다.
Bagging is one of the most effective procedure to improve the performance on unstable estimators or classifiers. It draws bootstrap sample, and then averages the resulting prediction rule. When using unstable classifier, bagging reduce the variance of a classifier. However, there is no guarantee that bagging will improve the performance of any base classifier. When using stable base classifiers, like support vector machines, it may even yield a deterioration of predictive accuracy. In this study, we propose new bagging algorithm to improve the performance of any classifiers. The first idea is to aggregate the bootstrapped classification rules that weight to reflect the predict accuracy. The second idea is similar previous one. Instead of averaging over all bootstrapped classifiers, we trim away those bootstrapped classifiers that result in the highest error rate. Afterward, we perform the same work previous procedure. On the basis of real data experiments, we conclude that idea proposed in this paper is performing well comparably to standard bagging when applied to unstable classifiers as decision trees. Moreover, it yields better results when applied to stable base classifiers, like support vector machines.
1. 서론
2. 배깅 방법론
3. 가중평균을 이용한 배깅 방법론
4. 실제자료의 적용
5. 결론 및 향후 연구과제
참고문헌
(0)
(0)