유사성 측도에 대한 연관성 규칙 평가 기준으로의 적용 방안
The Application of Some Similarity Measures to Association Rule Thresholds
- 한국자료분석학회
- Journal of The Korean Data Analysis Society (JKDAS)
- Vol.13 No.3
-
2011.061331 - 1341 (11 pages)
- 3
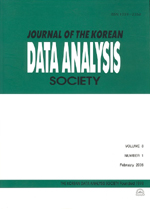
연관성 규칙은 방대한 양의 데이터베이스에 내재되어 있는 흥미로운 관계를 발견하기 위한 기법으로 주어진 데이터 집합에서 항목들간의 관계를 수치화하는 기법이다. 의미 있는 연관성 규칙을 탐사하기 위한 가장 기본적인 연관성 규칙 평가 기준에는 지지도, 신뢰도, 향상도 등이 있으며, 우리는 이들을 이용하여 의미 있는 연관성 규칙을 생성하게 된다. 이들 중에서 지지도와 향상도는 대칭적인 측도인 반면에 신뢰도는 비대칭적 측도이다. 비대칭 측도가 되면 전항과 후항이 바뀌게 되면 그 값이 달라지므로 이들 간에 관련성 여부를 판단하기가 곤란해진다. 본 논문에서는 이러한 문제를 해결하기 위해 군집분석이나 다차원 분석에서 활용되고 있는 동시 비 발생 빈도를 고려하지 않은 유사성 측도를 대칭적 연관성 평가 기준으로 이용하는 방안에 대해 연구하였다. 이러한 유사성 측도를 탐색한 후, 예제를 통하여 기존의 신뢰도와 비교함으로써 유사성 측도의 유용성을 알아보았다. 그 결과, 본 논문에서 고려한 유사성 측도들은 신뢰도의 단점을 보완할 수 있는 연관성 평가 기준으로 활용할 수 있다는 사실을 확인하였다.
Association rule mining is a popular and well researched method for discovering interesting relations among large set of data items. Association rule mining is the method to quantify the relationship between each set of items in a given dataset. There are three primary quality measures for association rules ; support and confidence and lift. We generate some meaningful association rules using these measures. Support and lift are symmetric measures for association rule exploration, but confidence is an asymmetric measure. If we use confidence for association rule generation, we cannot know whether the association is positive or negative by their values, and so we may reach the wrong conclusion. In this paper we apply some similarity measures not considering negative co-occurrence for binary data in cluster analysis and multi-dimensional analysis to association thresholds to compensate for these drawbacks. The comparative studies with support, confidence, and these similarity measures are shown by numerical example.
1. 서론
2. 유사성 측도의 탐색
3. 예제를 통한 유용성 고찰
4. 결론
참고문헌
(0)
(0)