NIG분포와 VG분포를 이용한 Value-at-Risk의 추정
Value-at-Risk Estimation using NIG and VG Distributions
- 한국자료분석학회
- Journal of The Korean Data Analysis Society (JKDAS)
- Vol.13 No.4
-
2011.081775 - 1788 (14 pages)
- 7
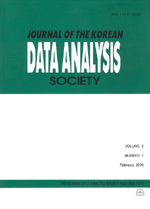
Value-at-Risk(VaR)를 구하기 위해 정규분포를 사용할 경우 추정은 간단하지만 실제로그수익률 분포의 꼬리가 정규분포보다 두텁기 때문에 VaR가 과소 추정될 수 있다. 본 논문에서는 대안적인 방법으로, 두터운 꼬리를 표현할 수 있으면서 금융자료를 설명하는 데에 자주 사용되는 normal inverse Gaussian(NIG)분포와 variance gamma(VG)분포를 이용하여 VaR를 추정해 보고자 한다. 이를 위해 KOSPI200 자료를 이용하여 NIG분포와 VG분포의 모수를 MLE방법으로 추정하고 VaR를 구하였다. 또, 정규분포를 가정하는 SMA방법과 EWMA방법, 역사적 시뮬레이션 방법, 그리고 극단치 이론을 이용하는 방법으로 VaR를 각각 추정한 후 사후검증을 통해 NIG분포와 VG분포를 이용한 VaR와 비교해 보았다. 그 결과 NIG분포를 이용했을 때 가장 좋은 성능을 보였으며 역사적 시뮬레이션 방법과 극단치 이론에 의한 방법도 우수한 성능을 보였다. 그러나 VG분포의 경우 모수추정이 불안정했고, VaR도 상대적으로 좋은 성능을 나타내지 못한 것으로 나타났다.
In this paper, we examine the performance of Value-at-Risk estimation methods using normal inverse gaussian (NIG) distribution and variance gamma (VG) distribution by comparing them with other existing methods. Since the distribution of financial asset return generally has fat tails and is sometimes skewed, methods of estimating VaR based on the normal distribution may cause a problem of underestimation. NIG and VG distributions are known to be able to represent some stylized facts of return distribution of financial assets. For the empirical study, we use log returns of KOSPI200 data of 10 years to compute VaR using several different methods. The result of backtesting reveals that the method based on NIG distribution has the best performance. However, VG model shows somewhat poor performance relative to other methods, possibly due to the unstable parameter estimation and the long computing time.
1. 서론
2. VaR의 개념과 추정방법
3. 실증분석
4. 결론
참고문헌
(0)
(0)