평점화 모형에서 입력변수의 절단값 선택 방법의 비교
A Comparison Study of Cutpoint Selection Methods in Credit Scoring
- 한국자료분석학회
- Journal of The Korean Data Analysis Society (JKDAS)
- Vol.13 No.6
-
2011.122915 - 2923 (9 pages)
- 13
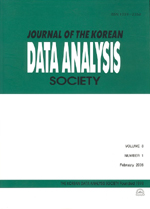
신용평점표 작성시 각 특성변수들을 적은 수의 속성들로 나누고 각 속성에 적절한 가중치를 부여하게 된다. 이러한 과정을 성김화라 한다. 성김화의 목적은 1) 신용대출에 대한 결정을 고객에게 이해하기 쉽도록 설명하기 위함이며 2) 신용위험도와 특성변수간에 비선형적 관계를 허용함으로써 예측력을 향상시키기 위한 것이다. 통계적 관점에서 보면 성김화는 연속형 변수를 몇 개의 구간으로 이산화하거나 이산형 변수의 수준들을 소수의 수준 그룹으로 재그룹화 하는 것이다. 본 논문에서는 연속형 변수를 이산화 할 때 그 기준이 되는 절단값 선택에 대하여 연구한다. 문헌상의 절단값 선택법으로는 등구간법, 분위수법, 모의 담금질법, 스플라인 분류 기계 등이 있다. 스플라인 분류 기계에서는 최적의 절단값들을 자동적으로 선택하는 반면 나머지 방법들은 절단값의 개수를 입력값으로 요구한다. 본 연구에서는 속성의 개수를 입력으로 요구하는 방법들에 대해서는 확인오차법으로 최적의 속성수와 절단값들을 동시에 결정한다. 모의실험 및 실제 신용자료 분석 결과 스플라인 분류 기계가 예측력과 계산속도의 측면에서 전반적으로 우수함을 알 수 있었다.
In constructing a credit scorecard, each characteristic variable is divided into a few attributes and weights are assigned to those attributes. Such a process is called coarse classification. The purposes of coarse classification are 1) to make a customer easily understand the decision on credit loan and 2) to improve a prediction by allowing a nonlinear relationship between the credit risk and a characteristic variable. From statistical perspective, coarse classification is to divide a continuous variable into a few intervals or regroup levels of a categorical variable into a small number of level groups. In this paper, we study the selection of cutpoints by which a continuous variable is discretized. In the literature, cutpoint selection methods such as equal spacing, quantile method, simulated annealing, and classification spline machine have been proposed. While classification spline machine automatically selects optimal cutpoints, the other methods requires the number of cutpoints as an input. We determine the optimal number of cutpoints by a cross-validation for those methods requiring the number of cutpoints as an input. Results from simulated and real credit data analysis indicate that overall performance of classification spline machine is good in terms of prediction and computing time.
1. 서론
2. 절단값 선택법
3. 자료 분석
4. 결론
참고문헌
(0)
(0)