편차정보기준을 이용한 베이지안 확률변동성 모형 선택에 관한 실증적 연구
Empirical Study on Bayesian Model Selection of Stochastic Volatility Models Using Deviance Information Criterion
- 한국자료분석학회
- Journal of The Korean Data Analysis Society (JKDAS)
- Vol.14 No.4
-
2012.081871 - 1888 (18 pages)
- 3
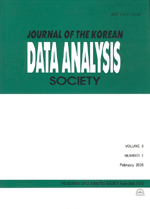
본 논문에서는 금융 시계열 자료의 변동성을 설명하기 위하여 베이지안 확률 변동성 모형을 고려하고 편차정보기준(DIC; deviance information criterion)을 바탕으로 확률변동성 모형에 대한 베이지안 모형 선택 문제를 연구한다. 이러한 베이지안 확률 변동성 모형을 적합하기 위한 마르코프 연쇄 몬테칼로(MCMC) 방법들을 설명하고, 이를 바탕으로 WinBUGS를 이용한 여러 가지 변동성 모형 하에서의 모형 적합과 각 모형에서의 편차정보기준을 계산하고 비교하는 실증적 연구를 수행한다. 구체적으로, 네 가지 베이지안 변동성 모형을 고려하고 이를 바탕으로 모의실험 자료를 생성하여 모형 적합과 편차정보기준 계산을 통한 모형 비교를 수행하였다. 모의실험자료 뿐만 아니라 S&P 500 지수와 KOSPI 자료를 바탕으로 한 사례연구를 통하여 확률변동성 모형 적합과 편차정보기준을 활용한 모형 선택에 대한 실증적 분석을 수행하였다.
In this paper, we consider Bayesian stochastic volatility models in order to explain the volatilities in the financial time series data and study Bayesian model selection of stochastic volatility models using deviance information criterion (DIC). We explain Markov chain Monte Carlo (MCMC) methods to estimate Bayesian stochastic volatility models, and perform empirical analysis by fitting various stochastic volatility models using WinBUGS, computing there DICs and comparing them. Specifically, numerical results on posterior estimation and DIC calculations are provided from empirical studies based on simulation data from four stochastic volatility models, and real applications using S&P index and KOSPI data are also considered for Bayesian model selection using DICs.
1. 서론
2. 베이지안 확률변동성 모형
3. 편차정보기준을 통한 베이지안 모형 선택
4. 결론
참고문헌
(0)
(0)