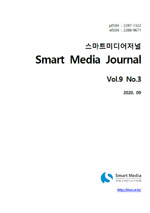
Removing Out - Of - Distribution Samples on Classification Task
- 한국스마트미디어학회
- 스마트미디어저널
- Vol9, No.3
- : KCI등재후보
- 2020.09
- 80 - 89 (10 pages)
Out - of - distribution (OOD) samples are frequently encountered when deploying a classification model in plenty of real-world machine learning-based applications. Those samples are normally sampling far away from the training distribution, but many classifiers still assign them high reliability to belong to one of the training categories. In this study, we address the problem of removing OOD examples by estimating marginal density estimation using variational autoencoder (VAE). We also investigate other proper methods, such as temperature scaling, Gaussian discrimination analysis, and label smoothing. We use Chonnam National University (CNU) weeds dataset as the in - distribution dataset and CIFAR-10, CalTeach as the OOD datasets. Quantitative results show that the proposed framework can reject the OOD test samples with a suitable threshold.
Ⅰ. INTRODUCTION
Ⅱ. PRELIMINARIES
Ⅲ. PROPOSED FRAMEWORK
Ⅳ. EXPERIMENT
Ⅴ. CONCLUSION
REFERENCES