기계학습 기법을 활용한 플립러닝 강좌의 LMS 로그파일 분석 사례 연구
Learning Management System Log Data Analysis via Machine Learning: A Case Study from Flipped Learning
- 한국열린교육학회
- 열린교육연구
- 열린교육연구 제28권 제5호
-
2020.1179 - 102 (24 pages)
-
DOI : 10.18230/tjye.2020.28.5.79
- 352
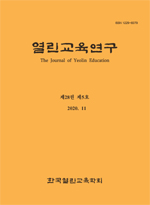
COVID-19 사태로 원격 수업은 이미 전세계적인 현상이 되며 온라인 자료를 활용한 학습분석학 연구의 중요성이 한층 더 강조되고 있다. 이러한 시대에 기계학습 기법을 활용하여 LMS (learning management system) 로그파일과 같은 빅데이터를 분석함으로써 학습자의 성공을 위한 예측모형을 구축할 필요가 있다. 온라인 학습에서 특히 자기조절학습이 중시되며 총 로그인 횟수 또는 평균 접속 시간과 같은 집계 변수(aggregate variable)로 모형에 활용되어 왔는데, 실제 학습시점에서의 자기조절학습 관련 변수를 측정하고 모형화할 필요가 있다. 본 연구의 목적은 플립러닝으로 이루어진 대학원 ‘기초통계’ 수업에서 학습자의 강의동영상 시청 양상을 파악하고, 기계학습 기법을 활용하여 학업성취도를 예측하는 변수를 탐색하는 것이었다. LMS 로그파일로부터 수업 전후 동영상 시청 빈도를 추출하고 학습자 배경 변수와 병합한 후 100개 설명변수를 활용하여 학업성취도 예측모형을 구축하였다. 본 연구는 기계학습 기법 중 벌점회귀모형으로 분류되는 Enet(elastic net)을 활용함으로써 상대적으로 해석하기 쉬운 예측모형을 도출하였다. 기존 연구 방식으로는 중간고사 또는 적어도 쪽지시험과 같은 평가 후에야 학습자의 수준을 가늠할 수 있었던 반면, 본 연구는 평가 자료 없이도 빠르게는 2~3주차부터 성취도를 예측하는 변수를 파악할 수 있었다. 사전학습 과제였던 조별 질문 생성이 학습자들의 사전학습을 효과적으로 촉진하였다는 것 또한 확인할 수 있었다. 단, 학습자의 배경지식이 깊지 않고 내용 자체가 어려운 강좌의 경우 무작정 강의동영상을 많이 보라고 학습자들을 독려하는 것이 적절하지 않을 수 있다. 대학원 수준 통계와 같은 상대적으로 어려운 강좌에서 플립러닝을 효과적으로 적용할 수 있는 방안 및 다양한 배경변인 및 학습 관련 변인을 수집하고 모형에서 활용하는 방안 등을 후속 연구과제로 논하였다.
The Learning Management System (LMS) provides big data for analysis with machine learning techniques to create prediction models for student success. In particular, self-regulated learning has been emphasized in online learning, and measured as login frequencies or login time. However, aggregate variables such as total login frequencies or average login time have been typically utilized, mainly due to analytical limitations of conventional techniques. More detailed indicators of self-regulated learning such as students’ watching frequencies of online lectures before and after each class need to be measured and modeled to predict students’ academic success via machine learning. This study aimed at exploring important variables for the prediction model as well as capturing students’ watching behaviors of online lectures. Students’ watching frequencies were coded using LMS log data from a graduate-level flipped classroom (FC). Combined with students’ background information, the final data for analysis consisted of 100 predictors in a course on introductory statistics. Enet (penalized regression) was employed among machine learning techniques mainly due to its interpretability. Without a quiz or an exam, the frequencies of video watching were important variables to predict students’ academic achievement as early as the first 2~3 weeks of the course. However, merely watching the instructional videos repeatedly did not contribute to students’ academic success. Further studies are warranted on designing effective FC with heavily loaded courses covering unfamiliar content as well as collecting and modeling other important study-related or background information variables relevant to students’ academic success in FC.
Ⅰ. 서론 및 연구의 필요성
Ⅱ. 선행 연구
Ⅲ. 벌점회귀모형
Ⅳ. 연구 방법
Ⅴ. 연구 결과
Ⅵ. 논 의
참고문헌
(0)
(0)