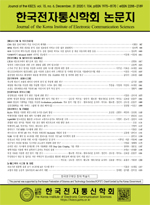
GOCI 영상과 기계학습 기법을 이용한 Cochlodinium polykrikoides 적조 탐지 기법 연구
Study on Detection for Cochlodinium polykrikoides Red Tide using the GOCI image and Machine Learning Technique
- 한국전자통신학회
- 한국전자통신학회 논문지
- 제15권 제6호
- : KCI등재
- 2020.12
- 1089 - 1097 (9 pages)
본 연구에서는 적조 Cochlodinium Polykrikoide를 기계학습 방법과 정지궤도 해색위성 영상을 활용하여 탐지하는 방법을 제안하다. 기계학습 모형을 학습시키기 위해 GOCI Level2 자료를 활용하였으며, 국립수산과학원의 적조 속보 자료를 활용하였다. 기계학습 모델은 로지스틱 회귀모형 , 의사결정나무 모형, 랜덤포레스트 모형을 사용하였다. 성능 평가 결과 기계학습을 사용하지 않은 전통적인 GOCI 영상 기반 적조 탐지 알고리즘 (Son et al.,2012) (75%)과 비교해보았을 때 약 13~22%p (88~98%)의 정헉도 향상을 확인할 수 있었다. 또한 기계학습 모형 간 탐지 성능을 비교 분석해본 결과 랜덤 포레스트 모형(98%)이 가장 높은 탐지 정확도를 보였다. 이러한 기계학습 기반 적조 탐지 알고리즘은 향후 적조를 조기에 탐지하고 그 이동과 확산을 추적 모니터링하는데 활용될 수 있을 것이라고 판단된다.
In this study, we propose a method to detect red tide Cochlodinium Polykrikoide using by machine learning and geostationary marine satellite images. To learn the machine learning model, GOCI Level 2 data were used, and the red tide location data of the National Fisheries Research and Development Institute was used. The machine learning model used logistic regression model, decision tree model, and random forest model. As a result of the performance evaluation, compared to the traditional GOCI image-based red tide detection algorithm without machine learning (Son et al., 2012) (75%), it was confirmed that the accuracy was improved by about 13~22%p (88~98%). Could. In addition, as a result of comparing and analyzing the detection performance between machine learning models, the random forest model (98%) showed the highest detection accuracy. It is believed that this machine learning-based red tide detection algorithm can be used to detect red tide early in the future and track and monitor its movement and spread.
Ⅰ. 서 론
Ⅱ. 자료 및 방법
Ⅲ. 결과 및 고찰
Ⅳ. 결 론
References