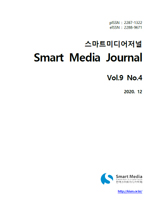
딥러닝 기반 이미지 특징 추출 모델을 이용한 유사 디자인 검출에 대한 연구
Detecting Similar Designs Using Deep Learning-based Image Feature Extracting Model
- 한국스마트미디어학회
- 스마트미디어저널
- Vol9, No.4
- : KCI등재후보
- 2020.12
- 162 - 169 (8 pages)
디자인은 섬유패션 산업에서 제품의 경쟁력을 결정짓는 핵심요인이다. 무단복제를 방지하고 독창성을 확인하기 위하여 제시된 디자인의 유사도를 측정하는 것은 매우 중요하다. 본 연구에서는 딥러닝 기법을 이용하여 섬유 디자인의 이미지로 부터 특징(feature)을 수치화하고, 스피어만 상관계수를 이용하여 유사도를 측정하였다. 유사한 샘플이 실제로 검출되는지 검증하기 위하여 300장의 이미지를 임의로 회전 및 색상을 변경하였다. 유사도 수치가 높은 순으로 Top-3와 Top-5의 결과에 회전을 하거나 색상을 변경한 샘플이 존재하는지 측정하였다. 그 결과, AlexNet 보다 VGG-16 모델이 월등히 높은 성능을 기록하였다. VGG-16 모델의 성능은 회전 이미지의 경우에 유사도 결과값이 높은 Top-3와 Top-5에서 64%, 73.67%로 가장 높게 나타났다. 색상변경의 경우에는 Top-3와 Top-5에서 각각 86.33%, 90%로 가장 높게 나타났다.
Design is a key factor that determines the competitiveness of products in the textile and fashion industry. It is very important to measure the similarity of the proposed design in order to prevent unauthorized copying and to confirm the originality. In this study, a deep learning technique was used to quantify features from images of textile designs, and similarity was measured using Spearman correlation coefficients. To verify that similar samples were actually detected, 300 images were randomly rotated and color changed. The results of Top-3 and Top-5 in the order of similarity value were measured to see if samples that rotated or changed color were detected. As a result, the VGG-16 model recorded significantly higher performance than did AlexNet. The performance of the VGG-16 model was the highest at 64% and 73.67% in the Top-3 and Top-5, where similarity results were high in the case of the rotated image. appear. In the case of color change, the highest in Top-3 and Top-5 at 86.33% and 90%, respectively.
Ⅰ. 서론
Ⅱ. 관련 연구
Ⅲ. 연구 방법론
Ⅳ. 연구 설계
Ⅴ. 연구 결과
Ⅵ. 결론
REFERENCES