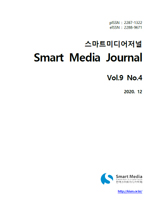
A Study on Applying the SRCNN Model and Bicubic Interpolation to Enhance Low-Resolution Weeds Images for Weeds Classification
- 한국스마트미디어학회
- 스마트미디어저널
- Vol9, No.4
- : KCI등재후보
- 2020.12
- 17 - 25 (9 pages)
In the image object classification problem, low-resolution images may have a negative impact on the classification result, especially when the classification method, such as a convolutional neural network (CNN) model, is trained on a high-resolution (HR) image dataset. In this paper, we analyze the behavior of applying a classical super-resolution (SR) method such as bicubic interpolation, and a deep CNN model such as SRCNN to enhance low-resolution (LR) weeds images used for classification. Using an HR dataset, we first train a CNN model for weeds image classification with a default input size of 128×128. Then, given an LR weeds image, we rescale to default input size by applying the bicubic interpolation or the SRCNN model. We analyze these two approaches on the Chonnam National University (CNU) weeds dataset and find that SRCNN is suitable for the image size is smaller than 80×80, while bicubic interpolation is convenient for a larger image.
I. INTRODUCTION
II. RELATED WORK
III. METHODOLOGY
IV. EXPERIMENTS
V. CONCLUSION
REFERENCES