선적분에 의한 위상차 영상의 줄무늬 아티팩트 감소를 위한 기계학습법에 대한 평가
Evaluation of Machine Learning Methods to Reduce Stripe Artifacts in the Phase Contrast Image due to Line-Integration Process
- 한국방사선학회
- 한국방사선학회 논문지
- 제14권 제7호
-
2020.12937 - 946 (10 pages)
-
DOI : 10.7742/jksr.2020.14.7.937
- 21
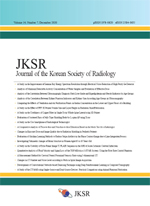
격자간섭계는 한 위상 물체에 의한 파두의 굴절변화로 인해 그 물체에 대한 미분 위상 영상을 제공하며, 이 미분 위상 영상은 위상 영상으로 전환되어야 할 필요가 있다. 미분 위상차 영상으로부터 위상차 영상을 얻기 위한 선적분 과정은 노이즈를 축적하고 줄무늬 아티팩트를 생성한다. 줄무늬 아티팩트는 선적분이 수행된 위상차 영상에서 적분 방향으로 노이즈와 왜곡이 증가한다. 이 연구에서는 이러한 아티팩트를 줄이기 위해 몇 가지 기계 학습 방법들을 구성하고 비교하였다. 기계 학습 방법들은 상호비교를 위하여 시뮬레이션 된 수치 팬텀과 엑스선 및 중성자 격자 간섭계로부터 얻어진 실험 데이터에 적용되었다. 그 결과 웨이블릿 전처리와 기계 학습 방법(WCNN)의 조합이 가장 효과적인 것으로 나타났다.
The grating interferometer provides the differential phase contrast image of an phase object due to refraction of the wavefront by the object, and it needs to be converted to the phase contrast image. The line-integration process to obtain the phase contrast image from a differential phase contrast image accumulates noise and generate stripe artifacts. The stripe artifacts have noise and distortion increases to the integration direction in the line-integrated phase contrast image. In this study, we have configured and compared several machine learning methods to reduce the artifacts. The machine learning methods have been applied to simulated numerical phantoms as well as experimental data from the X-ray and neutron grating interferometer for comparison. As a result, the combination of the wavelet preprocessing and machine learning method (WCNN) has shown to be the most effective.
Ⅰ. INTRODUCTION
Ⅱ. MATERIAL AND METHODS
Ⅲ. Results and Discussion
Ⅳ. CONCLUSION
Reference
(0)
(0)