딥러닝을 이용한 공간예측
Spatial Prediction using the Deep Learning
- 한국자료분석학회
- Journal of The Korean Data Analysis Society (JKDAS)
- Vol.23 No.1
-
2021.02149 - 160 (12 pages)
-
DOI : 10.37727/jkdas.2021.23.1.149
- 이용수 133
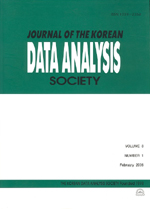
키워드
초록
공간상에서 얻어지는 데이터는 일반통계학과 다르게 얻어지는 관측값들은 서로 상관되어 있다는 전제하에서 분석이 행해진다. 공간통계학에서 공간데이터는 (1) 경험 베리오그램 추정, (2) 추정된 경험베리오그램을 이용한 이론베리오그램 적합, 그리고 (3) 이론베리오그램을 이용하여 기지(旣知)의 위치에서 측정된 관측값을 이용하여 미지(味知)의 위치에서의 관측값을 예측하는 크리깅의 과정을 거쳐 분석된다. 최근 이슈화되고 있는 인공지능 기법의 하나인 딥러닝이 역시 예측의 한 방법으로 널리 적용되고 있다. 전기한 두 방법 모두 예측을 한다는 측면에서는 유사 하지만, 공간통계학의 분석과정을 거쳐 예측하는 방법은 분석자의 주관이 개입될 수 있을 뿐만 아니라 분석과정은 그리 간단하지 않다. 그리고 공간데이터 분석의 가정을 만족하지 못하는 경우도 있다. 그러나 딥러닝은 이론적으로는 복잡할 수 있으나 공간통계학에서 행해지는 분석방법 보다 훨씬 사용하기가 간단하다는 장점이 있다. 본 연구에서는 시뮬레이션을 이용하여 두 방법을 사용하여 분석을 수행하고, 어느 방법이 더 예측적인 측면에서 우월한가에 대해서 알아보고, 실제 적용 예를 통해서 본 연구의 타당성을 알아본다. 예측력의 기준으로 RMSE를 사용하였고, 분석결과 기존의 공간통계학 예측방법과 딥러닝 기법에 따른 예측력은 비슷한 결과를 보였다. 따라서 공간데이터를 분석함에 있어 공간예측의 단점을 보완할 수 있는 딥러닝을 적용한 공간예측 분석을 제안한다.
Unlike general statistics, spatial data is analyzed on the premise that they are spatially correlated. In general, spatial data is analyzed by kriging method as follow stages: (1) Estimating an empirical variogram, (2) Fitting a theoretical variogram using the estimated empirical variogram, (3) Predicting the value in the unknown location using the observations from the known location with the fitted theoretical variogram. Deep learning, one of the artificial intelligence techniques that have recently become an issue, is also widely applied as a way of prediction. Although both methods are similar in terms of making predictions, not only can the subject of the analyst be involved in the method of predicting through the analysis process of kriging method, but the analysis process is not very simple. However, deep learning can be complex to design, but it has the advantage of being much simpler to use than analytical methods conducted in kriging method. In this work, we use both methods to conduct our analysis and to find out which methods are superior in terms of more spatial predictive aspects. The RMSE was used as the criteria for prediction performance. The result of the prediction comparison showed that kriging method and deep learning have similar power for spatial prediction. Therefore, we propose to perform spatial analysis by applying deep learning that can compensate for the disadvantages of spatial prediction by triple stages of kriging method.
목차
1. 서론
2. 공간통계학
3. 딥러닝
4. 시뮬레이션
5. 사례분석
6. 결론
References
참고문헌 (0)
등록된 참고문헌 정보가 없습니다.
해당 권호 수록 논문 (0)
등록된 수록 논문 정보가 없습니다.