서포트 벡터 기계에서 메타 학습법에 대한 연구
Proposal of Meta-Learning in Support Vector Machines
- 한국자료분석학회
- Journal of The Korean Data Analysis Society (JKDAS)
- Vol.23 No.1
-
2021.02125 - 134 (10 pages)
-
DOI : 10.37727/jkdas.2021.23.1.125
- 29
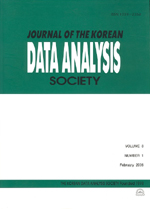
순차적 근사 최적화는 계산 비용이 많이 드는 함수들을 갖는 많은 공학 문제를 해결하기 위하여 적용되는 방법이다. 메타 모형(근사함수 형식)은 몇개의 표본을 기반으로 구성되며 한 번에몇 개의 표본을 추가하여 순차적으로 개선된다. 서포트 벡터 회귀(support vector regression) 및 방사형 기저 함수 네트워크(radial basis function networks)와 같은 계산 지능이 메타 모델화에 효과 적으로 적용될 수 있음이 알려져 왔다. 모형의 성능은 가우시안 함수의 폭 및 정규화 매개 변수와 같은 초모수(hyper-parameter)들의 영향을 받는다. 적합하거나 적절한 초모수들을 추정하는 가장 널리 사용되는 방법들 중에서 대표적인 방법은 교차 타당성 검정이다. 그러나 교차 타당성 검정은 일반적으로 시간이 많이 소요되며 표본점들의 수가 적은 순차적 근사 최적화에는 적용되지 않을 수 있다. 본 연구에서는 가우시안 함수의 폭을 선택하는 부담을 줄이고 가능한 적은 수의 표본들로 좋은 근사 모델을 생성하기 위하여 배깅(bagging)과 부스팅(boosting)을 사용하는 순차적 메타 학습 방법을 제안한다. 또한 제안된 방법의 효율성을 수치 실험을 통하여 일반화 능력과 모수의 조절이라는 관점에서 조사하였다.
Sequential approximate optimization is applied for solving many engineering problems with computationally expensive functions. Meta model (approximated function form) is constructed based on some samples and is improved in sequence by adding a few samples at a time. It has been observed that computational intelligence such as support vector regression(SVR) and radial basis function networks(RBFN) can be effectively applied to meta-modeling. The performance is affected by hyper-parameters, for example, Gaussian width and a regularization parameter. One of the most popular methods for estimating suitable/adequate hyper-parameters is the cross validation(CV) test. However, CV test is usually time consuming, and, may not be applicable for sequential approximation optimization with a small number of sample points. In this research, we suggest a sequential meta-learning method using bagging and boosting in order to reduce the burden on choosing the width of Gaussian function as well as to generate a good approximate model with as a small number of samples as possible. In addition, the effectiveness of the proposed method is investigated in terms of generalization ability and control of parameter through numerical experiments.
1. 서론
2. 서포트 벡터 회귀
3. 앙상블 학습
4. 수치실험
5. 결론
References
(0)
(0)