속산 시뮬레이션을 위한 적응형 비모수 중요 샘플링 기법
Non-parametric Adaptive Importance Sampling for Fast Simulation Technique
- 한국시뮬레이션학회
- 한국시뮬레이션학회 논문지
- 제8권 제3호
-
1999.0977 - 89 (13 pages)
- 0
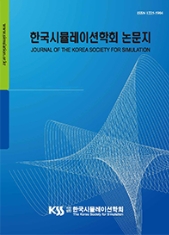
Simulating rare events, such as probability of cell loss in ATM networks, machine failure in highly reliable systems, requires huge simulation efforts due to the low chance of occurrence. Importance Sampling (IS) has been applied to accelerate the occurrence of rare events. However, it has a drawback of effective biasing scheme to make the estimator of IS unbiased. Adaptive Importance Sampling (AIS) employs an estimated sampling distribution of IS to the system of interest during the course of simulation. We propose Nonparametric Adaptive Importance Sampling (NAIS) technique which is nonparametrical version of AIS. We test NAIS to estimate a probability of rare event in M/M/1 queueing model. Comparing with classical Monte Carlo simulation, the computational efficiency and variance reductions gained via NAIS are substantial. A possible extension of NAIS regarding with random number generation is also discussed.
1. 서론
2. 전통적 Monte Carlo 시뮬레이션과 중요 샘플링 (Importance Sampling) 기법
3. 비모수 중요샘플링(Nonparametric Importance Sampling)
4. 적응형 중요샘플링
5. 적응형 비모수 중요샘플링(Non-parametric Adaptive Importance Sampling)
6. 적응형 비모수 중요샘플링 기법의 M/M/1 대기행렬에의 적용
7. 결론
참고문헌
(0)
(0)