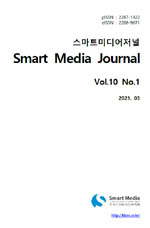
Few-Shot Image Synthesis using Noise-Based Deep Conditional Generative Adversarial Nets
- 한국스마트미디어학회
- 스마트미디어저널
- Vol10, No.1
- : KCI등재후보
- 2021.03
- 79 - 87 (9 pages)
In recent years research on automatic font generation with machine learning mainly focus on using transformation-based methods, in comparison, generative model-based methods of font generation have received less attention. Transformation-based methods learn a mapping of the transformations from an existing input to a target. This makes them ambiguous because in some cases a single input reference may correspond to multiple possible outputs. In this work, we focus on font generation using the generative model-based methods which learn the buildup of the characters from noise-to-image. We propose a novel way to train a conditional generative deep neural model so that we can achieve font style control on the generated font images. Our research demonstrates how to generate new font images conditioned on both character class labels and character style labels when using the generative model-based methods. We achieve this by introducing a modified generator network which is given inputs noise, character class, and style, which help us to calculate losses separately for the character class labels and character style labels. We show that adding the character style vector on top of the character class vector separately gives the model rich information about the font and enables us to explicitly specify not only the character class but also the character style that we want the model to generate.
I. INTRODUCTION
II. RELATED WORKS
III. PROPOSED METHOD
IV. EXPERIMENTS
V. DISCUSSION
VI. CONCLUSION