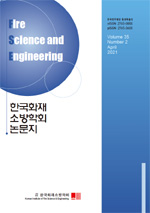
HSV 컬러 모델 및 코너 검출 알고리즘을 이용한 딥러닝 기반의 화염 감지에 관한 연구
Flame Detection Based on Deep Learning Using HSV Color Model and Corner Detection Algorithm
- 한국화재소방학회
- 한국화재소방학회논문지
- Vol.35 No.2
- : KCI등재
- 2021.04
- 108 - 114 (7 pages)
최근 딥러닝 기법을 이용한 이미지 분류 모델이나 객체 감지 모델이 많이 연구되고 있지만 적절한 전처리 방법을 설계하지 않을 경우 성능 평가 결과 낮은 정확도를 얻을 수 있다. 따라서 본 연구에서 제안하는 효과적인 화염검출전처리 방법으로는 HSV 컬러 모델과 Harris 코너 검출 알고리즘을 적용한 이미지 전처리 방법이다. HSV 컬러 모델을 통해 화염이 존재하는 색상영역을 필터링하고, 필터링된 결과물에 대해 Harris 코너 검출 방법을 적용할 경우 화염 이미지의 거친 질감 특성 때문에 화염 주변에 집중적으로 코너가 검출되게 된다. 이러한 특성을 통해 코너가 다수 발생한 영역을 관심영역으로 검출하여 딥러닝 기반의 합성곱신경망(Convolutional neural network, CNN) 모델을 통해 최종적으로 화염 여부를 분류하도록 하였다. 그 결과 본 연구에서 제안한 모델의 화염 검출 결과 정확도는 97.5% 정밀도는 97%로 나타났다.
Recently, many image classification or object detection models that use deep learning techniques have been studied; however, in an actual performance evaluation, flame detection using these models may achieve low accuracy. Therefore, the flame detection method proposed in this study is image pre-processing with HSV color model conversion and the Harris corner detection algorithm. The application of the Harris corner detection method, which filters the output from the HSV color model, allows the corners to be detected around the flame owing to the rough texture characteristics of the flame image. These characteristics allow for the detection of a region of interest where multiple corners occur, and finally classify the flame status using deep learning-based convolutional neural network models. The flame detection of the proposed model in this study showed an accuracy of 97.5% and a precision of 97%.
1. 서론
2. 이미지 전처리 방법 설계
3. 딥러닝 기반의 합성곱신경망 설계
4. 실험결과 및 성능평가
5. 결론