함수형 자료 기법을 활용한 평균 기온의 변화점 분석
Change Point Analysis for Average Temperature using Functional Data Methods
- 한국자료분석학회
- Journal of The Korean Data Analysis Society (JKDAS)
- Vol.23 No.4
-
2021.081629 - 1640 (12 pages)
-
DOI : 10.37727/jkdas.2021.23.4.1629
- 110
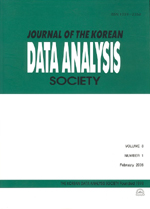
전 지구적 현상으로 평균기온이 상승해오고 있는 것으로 알려져 있다. 우리나라 평균기온 역시 상승해오고 있기 때문에 부산과 인근 지역 평균기온 변화 패턴도 크게 다르지 않을 것으로 생각된다. 그러나 어떤 시점부터 평균기온이 상승했는가 하는 질문에는 쉽게 답을 할 수 없을 것이다. 기온을 비롯한 기후요소들은 서서히 영향을 받아 변화하는 특성 때문에 변화했다는 것은 인지 할 수 있지만 어떤 시점에서 급진적인 변화가 이루어졌는가를 판단하는 연구는 많지 않은 편이다. 본 연구에서 실제적으로 부산 인근 지역의 평균기온의 변화 시점을 발견하는 데 초점을 맞추고자 한다. 구체적으로 기상청의 자동화 장치에 의하여 수집된 평균기온 자료를 기존에 널리 사용되어온 시계열 분석 방법이 아니라 함수형 자료(functional data) 관점에서 분석하고자 하며 분석 결과를 통해 시계열 분석 방법과의 차이점을 확인한다. 함수형 자료 관점에서 분석하기 위해 각 자료에 대한 기저 함수를 유도하며 함수형 자료 분석(functional data analysis)에서 자주 사용되는 functional principal components(FPCs) 추정하고 이에 기반 한 변화점 탐지 모형을 적용하여 자료를 분석한다. 그 결과 변화점 이후의 추세와 이상치로 취급할 수 있는 특정 년도를 발견하였다.
It is known that the average temperature has been increased as a global phenomenon. Since the average temperature in Korea has also been increased, it is expected that the pattern of changes in the average temperature in Busan and neighboring areas will not differ significantly. However, the question of when the average temperature has increased cannot be easily answered. It can be easily recognized that climate factors including temperature, have changed due to the characteristics that are gradually changed, but there are not many studies that determine at what point the radical change occurred. In this paper, we will focus on finding the time of change of the average temperature in Busan. Specifically, we intend to analyze the average temperature data collected by the Meteorological Agency s automated device by treating them as functional objects, not as time series. And we confirm the difference of result between functional data analysis and time series analysis. In order to derive the basis function for each data, we estimate functional principal components, which are often used in functional data analysis, and analyze the data by applying a change point detection model. As a result, we found a particular year that could be treated as an outlier and a trend after the change point.
1. 서론
2. 분석방법
3. 자료 분석
4. 결론
References
(0)
(0)