Prelude to Machine Learning-Based IRT Research: Bayesian Item Parameter Recovery
- 한국자료분석학회
- Journal of The Korean Data Analysis Society (JKDAS)
- Vol.23 No.4
-
2021.081509 - 1516 (8 pages)
-
DOI : 10.37727/jkdas.2021.23.4.1509
- 27
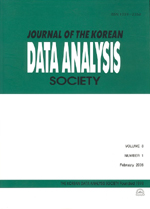
Using the complimentary software R and WinBugs, this study examined the item parameter recovery via Markov chain Monte Carlo (MCMC) and its convergence diagnostic measures. Ten sets of dichotomous response data were generated under the condition of 1000 examinees and 21 items with the simplest item response theory (IRT) model (that is, one parameter logistic model (1-PLM)). This study followed four steps: (1) generating 10 replication data sets using R, (2) calibrating 1-PL IRT model to those artificial data sets with WinBugs, (3) checking convergence measures via the specialized diagnostic tool, R package ‘boa’, and (4) evaluating parameter recovery performance by examining a sort of summary statistics such as RMSE. The present study shed light on the Bayesian IRT framework and the performance of statistical software in terms of item parameter recovery. Plus, by employing a series of education big data, this paper serves as a prelude to subsequent machine learning-based IRT research.
1. Introduction
2. Method
3. Results
4. Concluding remarks
References
(0)
(0)