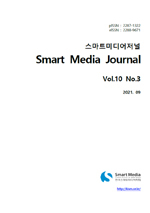
부분복제 지수 상향 추종을 위한 진화 알고리즘 기반 3단계 포트폴리오 선택 앙상블 학습
3-stage Portfolio Selection Ensemble Learning based on Evolutionary Algorithm for Sparse Enhanced Index Tracking
- 한국스마트미디어학회
- 스마트미디어저널
- Vol10, No.3
- : KCI등재후보
- 2021.09
- 39 - 47 (9 pages)
지수 상향 추종은 시장수익률을 좇는 지수 추종에 기반을 두고 지수 이상의 수익을 내기 위한 목적함수를 최적화시키는 문제이다. 큰 거래비용과 비유동성 등의 문제를 피하기 위하여 지수 편입종목들 중 일부만을 선택하여 포트폴리오를 구성하는 방법을 사용하였다. 기존의 지수 상향 추종 방법들은 테스트하는 모든 구간에서 하나의 목적함수만을 가지고 최적의 포트폴리오를 찾고자 하였으나 변동성이 큰 금융시장에서 항상 잘 통하는 궁극의 전략을 찾고자 하는 것은 불가능에 가깝다. 또한, 시간에 따라 통계적 특성이 크게 변하는 금융시장의 특성 상 학습 데이터에 대한 목적함수를 최적화시키는 것 이상으로 일반화 성능을 향상시키는 것이 중요하지만 기존의 방법들은 이를 위한 직접적인 논의가 부족하다는 한계점을 가지고 있다. 이러한 문제점들을 해결하기 위하여 본 논문은 여러 목적함수들을 조합하여 포트폴리오를 구성하는 앙상블 학습과 학습 데이터에 대한 목적함수 이외의 다른 기준을 적용하여 포트폴리오를 선택할 수 있는 3단계 포트폴리오 선택 알고리즘을 제안한다. S&P500 지수를 사용한 실험에서 제안한 방법은 지수와 기존 방법들보다 27% 이상 높은 Sharpe ratio를 보이며 3단계 포트폴리오 선택 알고리즘과 앙상블 학습이 지수 상향 포트폴리오를 선택하는데 효과가 있음을 보여준다.
Enhanced index tracking is a problem of optimizing the objective function to generate returns above the index based on the index tracking that follows the market return. In order to avoid problems such as large transaction costs and illiquidity, we used a method of constructing a portfolio by selecting only some of the stocks included in the index. Commonly used enhanced index tracking methods tried to find the optimal portfolio with only one objective function in all tested periods, but it is almost impossible to find the ultimate strategy that always works well in the volatile financial market. In addition, it is important to improve generalization performance beyond optimizing the objective function for training data due to the nature of the financial market, where statistical characteristics change significantly over time, but existing methods have a limitation in that there is no direct discussion for this. In order to solve these problems, this paper proposes ensemble learning that composes a portfolio by combining several objective functions and a 3-stage portfolio selection algorithm that can select a portfolio by applying criteria other than the objective function to the training data. The proposed method in an experiment using the S&P500 index shows Sharpe ratio that is 27% higher than the index and the existing methods, showing that the 3-stage portfolio selection algorithm and ensemble learning are effective in selecting an enhanced index portfolio.
Ⅰ. 서론
Ⅱ. 배경지식
Ⅲ. 관련 연구
Ⅳ. 연구 동기 및 기여
Ⅴ. 제안 방법
Ⅵ. 실험
Ⅶ. 결론