Machine Learning Techniques with Item Response Data
- 한국자료분석학회
- Journal of The Korean Data Analysis Society (JKDAS)
- Vol.23 No.5
-
2021.102011 - 2023 (13 pages)
-
DOI : 10.37727/jkdas.2021.23.5.2011
- 58
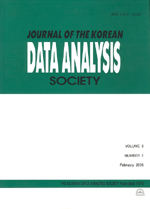
This is a two-pronged study. Two aims of this study are both interested in comparison of 1) different R IRT (Item Response Theory) packages and 2) different machine learning techniques. The first part of the present study addresses the performance of a couple of psychometric software available in R. Then, using item parameters from real data sets from the Program of International Student Assessment 2015, three machine learning techniques (classification tree, random forest, naïve Bayes) are assessed in the second part. Between these two parts, connections of IRT and ML are briefly described and reviewed with reference. Findings are 1) two comparisons of two sets of packages for each IRT model are not informative (from the part I) and 2) the classifiers of random forest and naïve Bayes algorithms performed better than the classification tree, which confirms that more information leads to more accurate prediction and ensemble approaches are better than single tree approaches. (from the part II). Finally, implications and caveats of this study are provided.
1. Introduction
2. R Item Response Theory (IRT) software and Machine Learning (ML)
3. Methods
4. Results
5. Conclusion
References
(0)
(0)