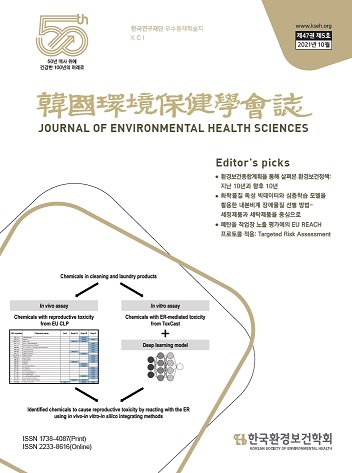
화학물질 독성 빅데이터와 심층학습 모델을 활용한 내분비계 장애물질 선별 방법: 세정제품과 세탁제품을 중심으로
A Screening Method to Identify Potential Endocrine Disruptors Using Chemical Toxicity Big Data and a Deep Learning Model with a Focus on Cleaning and Laundry Products
- 한국환경보건학회
- 1. 한국환경보건학회지
- 제47권 제5호
- : KCI우수등재
- 2021.10
- 462 - 471 (10 pages)
Background: The number of synthesized chemicals has rapidly increased over the past decade. For many chemicals, there is a lack of information on toxicity. With the current movement toward reducing animal testing, the use of toxicity big data and deep learning could be a promising tool to screen potential toxicants. Objectives: This study identified potential chemicals related to reproductive and estrogen receptor (ER)- mediated toxicities for 1135 cleaning products and 886 laundry products. Methods: We listed chemicals contained in cleaning and laundry products from a publicly available database. Then, chemicals that potentially exhibited reproductive and ER-mediated toxicities were identified using the European Union Classification, Labeling and Packaging classification and ToxCast database, respectively. For chemicals absent from the ToxCast database, ER activity was predicted using deep learning models. Results: Among the 783 listed chemicals, there were 53 with potential reproductive toxicity and 310 with potential ER-mediated toxicity. Among the 473 chemicals not tested with ToxCast assays, deep learning models indicated that 42 chemicals exhibited ER-mediated toxicity. A total of 13 chemicals were identified as causing reproductive toxicity by reacting with the ER. Conclusions: We demonstrated a screening method to identify potential chemicals related to reproductive and ER-mediated toxicities utilizing chemical toxicity big data and deep learning. Integrating toxicity data from in vivo , in vitro , and deep learning models may contribute to screening chemicals in consumer products.
I. 서 론
II. 연구방법
III. 결 과
IV. 고 찰
V. 결 론