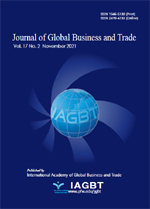
Credit Risk Measurement Study of Commercial Banks Based on the Innovation Discrete Hopfield Neural Network Model
- The International Academy of Global Business and Trade
- Journal of Global Business and Trade
- Vol. 17, No. 2
- 2021.11
- 13 - 27 (15 pages)
Purpose – Credit risk is always the major risk in the banking industry, and the main target of regulatory authorities. Based on modern financial theory and new credit instruments, modern risk measurement models have played a great role in the measurement of credit risk. However, most of these models assume normal distribution, which will lead to a deviation between the analysis results and the actual distribution of credit risk, c ause the “ thick tail” phenomenon, a nd reduce the accuracy of measurement results. In this context, the development of modern information technology makes it possible to introduce artificial intelligence technology into credit risk measurement, s uch as an artificial neural network, d ecision tree, g enetic programming, and support vector machine. Design/Methodology/Approach – Firstly, we use the commonly used an expert survey method and the research results and experience of previous scholars for reference to realize the transformation of regulatory indicators f rom qualitative t o quantitative. T hen, a c redit r isk measurement model based on t he Hopfield network was constructed in order to evaluate credit risk. Finally, the simulation experiment was carried out using real data from a commercial bank. Findings – The results show that a credit risk measurement model based on Hopfield neural network can accurately reflect the credit risk state of banking institutions. This shows that the credit risk measurement model proposed in this paper not only has the function of supporting decision-making for commercial bank managers, it is also an effective way for financial regulators to grasp the risk change trend in time. Research Implications – A credit risk measurement model of commercial banks based on a discrete Hopfield neural network is proposed. Because an artificial neural network has the advantages of strong “robustness”, high prediction accuracy, and is unstructured, this paper uses the associative memory function of DHNN to construct a credit risk measurement model based on a discrete Hopfield neural network. Simulation results show that the model can accurately reflect the credit risk status of banks.
Ⅰ. Introducton
Ⅱ. Discrete Hopfield Neural Network Method
Ⅲ. Empirical Analysis of the Credit Risk Measurement of Commercial Banks in China
Ⅳ. Conclusions