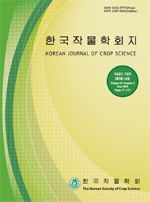
Identifying Factors for Corn Yield Prediction Models and Evaluating Model Selection Methods
Early predictions of crop yields can provide information to producers to take advantages of oppor-tunities into market places, to assess national food security, and to provide early food shortage warning. The objectives of this study were to identify the most useful parameters for estimating yields and to compare two model selection methods for finding the best model developed by multiple linear regression. This research was conducted in two 65-ha corn/soybean rotation fields located in east central South Dakota. Data used to develop models were small temporal variability information (STVI: elevation, apparent electrical conductivity (ECa), slope), large temporal varia-bility information (LTVI: inorganic N, Olsen P, soil mois-ture), and remote sensing information (green, red, and NIR bands and normalized difference vegetation index (NDVI), green normalized difference vegetation index (GDVI)). Second order Akaikes Information Criterion (AICc) and Stepwise multiple regression were used to develop the best-fitting equations in each system (information groups). The models with Di??2 were selected and 22 and 37 models were selected at Moody and Brookings, respectively. Based on the results, the most useful variables to estimate corn yield were different in each field. Elevation and ECa were consistently the most useful variables in both fields and most of the systems. Model selection was different in each field. Different number of variables were selected in different fields. These results might be contributed to different landscapes and management histories of the study fields. The most common variables selected by AICc and Stepwise were different. In validation, Stepwise was slightly better than AICc at Moody and at Brookings AICc was slightly better than Stepwise. Results suggest that the AICc approach can be used to identify the most useful information and select the best yield models for production fields.
MATERIALS AND METHODS
RESULTS AND DISCUSSION
REFERENCES