ANN 기반 도시침수 위험기준 예측 모델 개발 및 학습자료의 확장에 따른 영향 평가
Development of an ANN-Based Urban Flood Alert Criteria Prediction Model and the Impact of Training Data Augmentation
- 한국방재학회
- 2. 한국방재학회 논문집
- 21권6호
-
2021.12257 - 264 (8 pages)
-
DOI : 10.9798/KOSHAM.2021.21.6.257
- 149
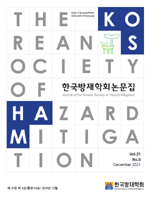
도시침수는 단기간 높은 강도의 집중호우에 의해 단시간에 발생하기 때문에 대응을 위해서는 신속하고 정확한 침수 예⋅경보가 필요하다. 선행연구에서 침수피해 통계기반의 도시침수 위험기준 산정 방법을 제시하고 침수 위험기준 예측을 위한 Neuro-Fuzzy 모델을 개발하였다. 최근 인공지능 기술의 발달로 다양한 인공지능 알고리즘의 활용도와 예측 정확성이 향상됨에 따라 도시침수 위험기준 예측에 적용하여 침수 위험기준의 정확성을 향상시키고자 한다. 따라서 본 연구에서는 Artificial Neural Network (ANN) 알고리즘을 이용하여 침수 위험기준을 예측하고;학습자료의 확장 기법 적용을 통한 영향을 분석하였다. ANN 모델의 예측 성능은 RMSE 3.39~9.80 mm;학습자료 확장을 통해 모델 성능은 RMSE 1.08~6.88 mm로 29.8~82.6% 개선되는 것을 확인하였다.
Urban flooding occurs during heavy rains of short duration;so quick and accurate warnings of the danger of inundation are required. Previous research proposed methods to estimate statistics-based urban flood alert criteria based on flood damage records and rainfall data;and developed a Neuro-Fuzzy model for predicting appropriate flood alert criteria. A variety of artificial intelligence algorithms have been applied to the prediction of the urban flood alert criteria;and their usage and predictive precision have been enhanced with the recent development of artificial intelligence. Therefore;this study predicted flood alert criteria and analyzed the effect of applying the technique to augmentation training data using the Artificial Neural Network (ANN) algorithm. The predictive performance of the ANN model was RMSE 3.39-9.80 mm;and the model performance with the extension of training data was RMSE 1.08-6.88 mm;indicating that performance was improved by 29.8-82.6%.
1. 서론
2. 연구내용 및 방법
3. 도시침수 위험기준 예측 모델 개발
4. 학습자료 확장에 따른 영향 분석
5. 결론
(0)
(0)