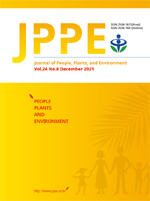
Comparison of Machine Learning Analysis on Predictive Factors of Children’s Planning-Organizing Executive Function by Income Level: Through Home Environment Quality and Wealth Factors
- 인간식물환경학회
- 인간식물환경학회지(JPPE)
- 제24권 제6호
- : SCOPUS, KCI등재
- 2021.12
- 651 - 662 (12 pages)
Background and objective: This study identifies whether children s planning-organizing executive function can be significantly classified and predicted by home environment quality and wealth factors. Methods: For empirical analysis, we used the data collected from the 10th Panel Study on Korean Children in 2017. Using machine learning tools such as support vector machine (SVM) and random forest (RF), we evaluated the accuracy of the model in which home environment factors classify and predict children s planning-organizing executive functions, and extract the relative importance of variables that determine these executive functions by income group. Results: First, SVM analysis shows that home environment quality and wealth factors show high accuracy in classification and prediction in all three groups. Second, RF analysis shows that estate had the highest predictive power in the high-income group, followed by income, asset, learning, reinforcement, and emotional environment. In the middleincome group, emotional environment showed the highest score, followed by estate, asset, reinforcement, and income. In the low-income group, estate showed the highest score, followed by income, asset, learning, reinforcement, and emotional environment. Conclusion: This study confirmed that home environment quality and wealth factors are significant factors in predicting children’s planning-organizing executive functions.
Introduction
Research Methods
Results and Discussion
Conclusion