Robust Interval Estimation Using Density Power Divergence
- 한국자료분석학회
- 한국자료분석학회 학술대회자료집
- 2021년 동계학술대회 발표집
-
2022.0147 - 51 (5 pages)
- 이용수 8
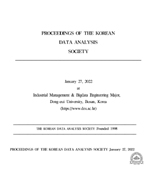
키워드
초록
It is well known that the maximum likelihood estimator (MLE) is asymptotically efficient but not robust with respect to both model misspecification and outliers. Basu et al. (1998) suggest a family of density-based divergence measures called `density power divergences . Each measure in this family is indexed by a single tuning parameter α, which controls the trade-off between robustness and asymptotic efficiency of the estimators. The Kullback-Leibler divergence (Kullback, Leibler, 1951) and 𝐿₂ -distance are members of this family. With a suitably chosen tuning parameter, a minimum density power divergence estimator (MDPDE) can be obtained. For 0<α<1, the estimator is in between MLE (efficient-but-nonrobust) and minimum 𝐿₂ -distance estimator 𝐿₂𝐸 (robust-but-inefficient). Hong, Kim(2001) suggest a data-driven selection of α. In this paper we will suggest a confidence interval using MDPDE when the data set is contaminated. Bootstrap resampling will be used to obtain the confidence interval. The resulting confidence intervals (called MDPD bootstrap confidence intervals) are expected to be robust with respect to the outliers. The performance of the MDPDE bootstrap confidence intervals are investigated via simulation study.
목차
Ⅰ. Introduction
Ⅱ. Robust confidence intervals using density power divergence
Ⅲ. Simulation Study
Ⅳ. Concluding Remarks
참고문헌 (0)
등록된 참고문헌 정보가 없습니다.
해당 권호 수록 논문 (0)
등록된 수록 논문 정보가 없습니다.