학술대회자료
Hidden Markov models for PM10 concentrations in Seoul
- 한국자료분석학회
- 한국자료분석학회 학술대회자료집
- 2021년 동계학술대회 발표집
-
2022.0131 - 34 (4 pages)
- 23
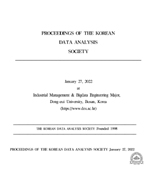
This study addresses the problem of monitoring and forecasting of particulate matter(PM) data, focusing, in particular, on high-level PM10 , which is known to adversely impact human mortality and morbidity. We use hourly PM10 data, collected over a period of 3 months between October 1, 2018, to December 31, 2018, from 40 stations located in the Seoul metropolitan area of South Korea. We model the number of regions corresponding to “bad” or “very bad” categories of the  density, using a hidden Markov model with Poisson state-dependent distribution, Poisson-HMM, since a Poisson-HMM allows for both overdispersion and serial dependence.
Ⅰ. Introduction
Ⅱ. Data
Ⅲ. Hidden Markov Models
Ⅳ. Data Analysis
References
(0)
(0)