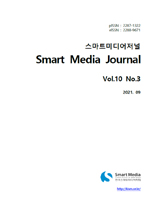
FPGA상에서 스파이킹 뉴럴 네트워크 지원을 위한 모델 최적화
Model Optimization for Supporting Spiking Neural Networks on FPGA Hardware
- 한국스마트미디어학회
- 스마트미디어저널
- Vol11, No.2
- : KCI등재후보
- 2022.03
- 70 - 76 (7 pages)
클라우드 서버를 이용한 IoT 응용 개발은 네트워크로 연결된 하드웨어에 데이터 송수신 지연, 네트워크 트래픽, 실시간 처리 지원을 위한 비용 등의 문제가 발생한다. 엣지 클라우드 기반 플랫폼에서는 이러한 문제를 해결하기 위해 빠른 데이터 전달이 가능하도록 뉴로모픽 하드웨어를 사용할 수 있다. 본 논문에서는 FPGA상에서 스파이킹 뉴럴 네트워크를 위한 모델 최적화 기법을 제안한다. 뉴로모픽 하드웨어에 최적화된 네트워크 모델 파라미터를 자동 조정하는 것에 초점을 맞추었다. 정확도에 대한 사용자 요구사항을 기반으로 더 높은 성능을 보이도록 최적화를 수행한다. 성능 분석 결과, 기존의 오픈 프레임워크에서 지원하는 고정 기법과 달리 사용자의 요구사항을 모두 만족하였으며 수행시간 측면에서 더 높은 성능을 보였다.
IoT application development using a cloud server causes problems such as data transmission and reception delay, network traffic, and cost for real-time processing support in network connected hardware. To solve this problem, edge cloud-based platforms can use neuromorphic hardware to enable fast data transfer. In this paper, we propose a model optimization method for supporting spiking neural networks on FPGA hardware. We focused on auto-adjusting network model parameters optimized for neuromorphic hardware. The proposed method performs optimization to show higher performance based on user requirements for accuracy. As a result of performance analysis, it satisfies all requirements of accuracy and showed higher performance in terms of expected execution time, unlike the naive method supported by the existing open source framework.
Ⅰ. 서론
Ⅱ. 관련연구
Ⅲ. 컴포넌트 모델 최적화 기법
Ⅳ. 성능평가
Ⅴ. 결론