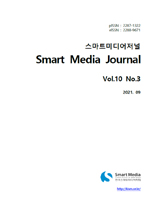
유튜브 악성 댓글 탐지를 위한 LSTM 기반 기계학습 시스템 설계 및 구현
Design and Implementation of a LSTM-based YouTube Malicious Comment Detection System
- 한국스마트미디어학회
- 스마트미디어저널
- Vol11, No.2
- : KCI등재후보
- 2022.03
- 18 - 24 (7 pages)
많은 소셜 서비스 상에서 악성 댓글로 인한 문제가 발생되고 있으며, 특히 매체로서의 성격이 강한 유튜브는 모바일기기를 이용한 쉬운 접근성으로 인해 악성 댓글로 인한 폐해가 더욱 커지고 있는 실정이다. 본 논문에서는 LSTM 기반의 자연어 처리를 통해 유튜브 콘텐츠에 대한 악성 댓글을 판별하고 악성 댓글의 비율, 악플러들의 닉네임, 그리고 빈도를 시각적으로 표현해 주기 위한 유튜브 악성 댓글 탐지 시스템을 설계하고 구현하였으며, 성능을 평가하였다. 약 5만 개의 댓글 데이터셋을 통해 악성 댓글 여부를 판별하였을 때, 약 92%의 정확도로 악성 댓글을 검출해 낼 수 있었으며, 이를 활용하여 악성 댓글의 통계가 자동으로 생성되도록 함으로써 많은 유튜버들이 겪는 악성 댓글로 인한 사회적 문제를 해결할 수 있을 것으로 기대한다.
Problems caused by malicious comments occur on many social media. In particular, YouTube, which has a strong character as a medium, is getting more and more harmful from malicious comments due to its easy accessibility using mobile devices. In this paper, we designed and implemented a YouTube malicious comment detection system to identify malicious comments in YouTube contents through LSTM-based natural language processing and to visually display the percentage of malicious comments, such commentors nicknames and their frequency, and we evaluated the performance of the system. By using a dataset of about 50,000 comments, malicious comments could be detected with an accuracy of about 92%. Therefore, it is expected that this system can solve the social problems caused by malicious comments that many YouTubers faced by automatically generating malicious comments statistics.
Ⅰ. 서론
Ⅱ. 연구 배경
Ⅲ. 본론
Ⅳ. 결론