LSTM Prediction of Streamflow during Peak Rainfall of Piney River
- 한국방재안전학회
- 한국방재안전학회 논문집
- 한국방재안전학회논문집 14권 4호
-
2021.1217 - 27 (11 pages)
-
DOI : 10.21729/ksds.2021.14.4.17
- 13
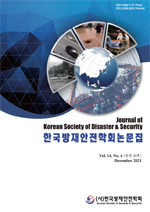
유량예측은 효과적인 홍수관리 및 수자원 계획을 위한 매우 중요한 재난방지 접근법이다. 현재 기후변화로 인한 집중호우가 나날이 증가하고 있어 막대한 기반시설 손실과 재산, 인명 피해가 발생하고 있다. 본 연구는 미국 테네시주 Hickman County의 Vernon에 있는 Piney Resort의 최근 홍수사례분석을 통해 최대 강우 시나리오에서 유량예측에 대한 강우의 기여도를 측정했다. Piney River 유역내 USGS 두개의 관측소(03602500, 03599500)에서 20년(2000-2019) 동안의 일별 하천 유량, 수위 및 강우 데이터를 수집했고, Long Short Term Memory(LSTM)을 사용하였다. 또한, Tensorflow, Keras Machine learning frameworks, Python을 이용하여 14일로 구별된 유량 값을 예측하였다. 또한, 모델이 2021년 8월 21일의 범람 이벤트를 예측할 수 있었는지를 결정하는 데 사용되었다. 전체 데이터(수위, 유량 및 강우량)가 포함된 LSTM 모델은 일부 강우 모델을 제외하고 지속성 모델보다 우수한 성능을 보였으며, 강우자료만 이용하여 유량예측을 하는 것은 충분하지 않음을 나타냈다. 결과는 LSTM 모델은 0.68 및 13.84m3/s의 최적 NSE 및 RMSE 값을 나타냈고, 가장 낮은 예측 오차로 예측 최대유량은 94m3/s로 나타났다. 향후 강우 패턴에 대한 다양한 분석이 이루어진다면 효율적인 홍수 경보 시스템 및 정책을 설계하는 관련 연구에 도움을 줄 것으로 판단된다.
Streamflow prediction is a very vital disaster mitigation approach for effective flood management and water resources planning. Lately, torrential rainfall caused by climate change has been reported to have increased globally, thereby causing enormous infrastructural loss, properties and lives. This study evaluates the contribution of rainfall to streamflow prediction in normal and peak rainfall scenarios, typical of the recent flood at Piney Resort in Vernon, Hickman County, Tennessee, United States. Daily streamflow, water level, and rainfall data for 20 years (2000-2019) from two USGS gage stations (03602500 upstream and 03599500 downstream) of the Piney River watershed were obtained, preprocesssed and fitted with Long short term memory (LSTM) model. Tensorflow and Keras machine learning frameworks were used with Python to predict streamflow values with a sequence size of 14 days, to determine whether the model could have predicted the flooding event in August 21, 2021. Model skill analysis showed that LSTM model with full data (water level, streamflow and rainfall) performed better than the Naive Model except some rainfall models, indicating that only rainfall is insufficient for streamflow prediction. The final LSTM model recorded optimal NSE and RMSE values of 0.68 and 13.84 m3/s and predicted peak flow with the lowest prediction error of 11.6%, indicating that the final model could have predicted the flood on August 24, 2021 given a peak rainfall scenario. Adequate knowledge of rainfall patterns will guide hydrologists and disaster prevention managers in designing efficient early warning systems and policies aimed at mitigating flood risks.
1. Introduction
2. Mathematical Background
3. Study Area
4. Methodology
5. Results and Discussion
6. Summary and Conclusion
References
(0)
(0)