비만 분류를 위한 인체 변수 선택 및 분류 방법에 대한 연구
Selection of Anthropometric Variables and Methods for Classification of Obesity
- 한국자료분석학회
- Journal of The Korean Data Analysis Society (JKDAS)
- Vol.24 No.2
-
2022.04613 - 625 (13 pages)
-
DOI : 10.37727/jkdas.2022.24.2.613
- 123
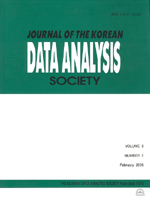
체질량지수(body mass index: BMI)는 적용이 편리하다는 장점이 있어 비만 판별에 가장 많이 활용되는 지표이다. 그러나 많은 선행연구에서 체질량지수로 비만을 분류한 결과가 체지방률을 기준으로 분류한 결과와 일치하지 않는다는 점이 지적되었고, 이는 미리 정의된 체질량지수 값을 기준으로 비만을 분류하는 것은 정확성이 떨어진다는 것을 의미한다. 따라서, 본 연구는 최소한의 신체 치수를 통해 비만을 판별할 수 있는 방법을 새로 제안하는 것을 목적으로 하였다. 이를 위해 기계학습 기법을 포함한 분류 방법을 적용하였고, 분류 성능을 정확도와 설명력 두 가지 측면에서 평가하였다. 분석 결과, 체질량지수와 체중을 활용한 퍼지 규칙 기반 시스템(fuzzy rule-based systems: FRBS)의 비만 분류 성능은 정확도 측면에서 다른 기계학습 알고리즘 및 판별분석과 같은 수준을 보임과 동시에 다른 모델 대비 더 일관된 분류 규칙을 제공하였기 때문에 비만 분류에 가장 적합하다는 결론을 얻었다. 따라서 본 연구는 FRBS 방법을 사용하여 도출한 분류 기준으로 비만을 판별할 것을 제안한다. 본 연구가 제안하는 방법은 체질량지수를 통한 기존의 비만 분류 방법만큼 편리하게 적용할 수 있으면서도 높은 분류 정확도를 보장하므로 비만분류 관련 연구에 기여를 할 수 있을 것으로 기대된다.
Body mass index(BMI) is an index commonly used for measuring body fat due to its convenience. However, many previous studies found that results of classification of obesity based on BMI and body fat percentage were not equivalent. This means the classification of obesity which refers to a predefined cutoff value of BMI may not be accurate. This study aims to suggest a better method for the classification of obesity using statistical methods and some AI techniques for classification which required a minimum number of input body dimensions. The classification performances were evaluated in terms of accuracy and interpretability. This study concluded BMI combined with weight is the best data set and suggests a fuzzy rule-based system (FRBS) as the most appropriate method for obesity classification. The FRBS model performed as accurately as other AI algorithms and determinant analysis and provided more consistent classification rules than others. Therefore, we propose to classify obesity using the FRBS method.
1. 서론
2. 방법
3. 결과
4. 결론 및 토의
References
(0)
(0)