Dimension Reduction and Classification with Weighted Supervised PCA
- 한국자료분석학회
- Journal of The Korean Data Analysis Society (JKDAS)
- Vol.24 No.2
-
2022.04485 - 495 (11 pages)
-
DOI : 10.37727/jkdas.2022.24.2.485
- 83
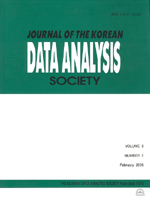
Supervised principal component analysis (PCA) is a useful dimension reduction method which is able to induce more discriminative features compared to the conventional PCA in regression or classification problems. This is because supervised PCA tries to extract principal directions by considering not only data variance in input space but also the information of output variable. In this paper, we introduce a simple weighted supervised PCA and apply it to a multi-category classification problem. The weights in the proposed method represent some importance or discriminative power of each input variable for classifying the labels of the output variable. Therefore, we modify directly the correlation matrix using the weights. PCA from this adjusted correlation matrix can produce principal directions that are more useful for classifying the output labels. Some data analyses verify that the proposed method is simple to apply and show good prediction accuracy.
1. Introduction
2. A Basic Review of PCA
3. Supervised PCA and Proposed Method
4. Data Analysis
5. Concluding Remarks
References
(0)
(0)