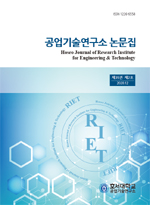
선박 탑승자 안전을 위한 양자화 딥러닝 기반 구명조끼 착용 판별에 관한 연구
A Study on the Identification of the Wearing of a Life Vest Based on Quantized Deep Learning for the Safety of Ship Passengers
- 호서대학교 공업기술연구소
- 공업기술연구 논문집
- 제39권 제2호
- 2020.12
- 39 - 47 (9 pages)
최근 인공지능 기술에 대해 관심이 증가함에 따라 디지털 영상에 특화되는 딥러닝 모델을 사용하는 연구가 활발히 진행되고 있다. 안테나를 이용한 구명조끼 관련 안전 연구는 사고가 발생한 후에 파악이 가능하지만 영상을 이용한 딥러닝을 적용하면 즉각적인 알람이 가능하여 선제적인 사고 예방이 가능하다. 그러나 딥러닝 모델의 복잡도가 높을수록 연산량이 급증하는 문제가 존재하므로 안전에 관련되는 시스템에서 딥러닝 모델은 실시간적인 처리가 매우 중요하다. 따라서 실시간 처리 및 상용화가 가능하려면 저비용의 프로세서에서도 동작할 수 있게 알고리즘을 경량화하는 기법이 필요하다. 따라서 본 논문에서는 선박 탑승자의 구명조끼 착용 여부를 판단하기 위해 모델의 훈련 및 추론에 사용되는 데이터를 전처리하고, 모델의 파라미터를 감소시키는 양자화 기법을 적용하여 메모리 용량을 절약하고 처리 속도를 향상하여 실시간적인 동작이 가능할 수 있도록 하는 방안을 제안하였다.
With the recent growing interest in Artificial Intelligence(AI) technologies, research using deep learning models that are specialized in digital images has been actively conducted. Safety studies related to life jackets using antennas can be identified after an accident, but applying deep learning using images enables immediate alarm, enabling preemptive accident prevention. However, in safety-related systems, real-time processing of deep learning models is critical, as the higher the complexity of deep learning models, the more computational problems exist. Therefore, for real-time processing and commercialization to be possible, techniques are needed to lighten the algorithm so that it can work even on low-cost processors. Therefore, this paper proposes to preprocess data used in training and inference of models to determine whether ship occupants wear life vests, and to apply quantization techniques that reduce the model s parameters to save memory capacity and improve processing speed to enable real-time operation.
I. 서론
II. 관련 연구
III. 본론
IV. 실험 결과
V. 결론