귀밑샘 암종에서 생존 예측을 위한 임상병리 인자 분석 및 머신러닝 모델의 구축
Clinico-pathologic Factors and Machine Learning Algorithm for Survival Prediction in Parotid Gland Cancer
- 대한두경부종양학회
- 대한두경부종양학회지
- 대한두경부종양학회지 제38권 제1호
-
2022.0517 - 24 (8 pages)
-
DOI : 10.21593/kjhno/2022.38.1.17
- 4
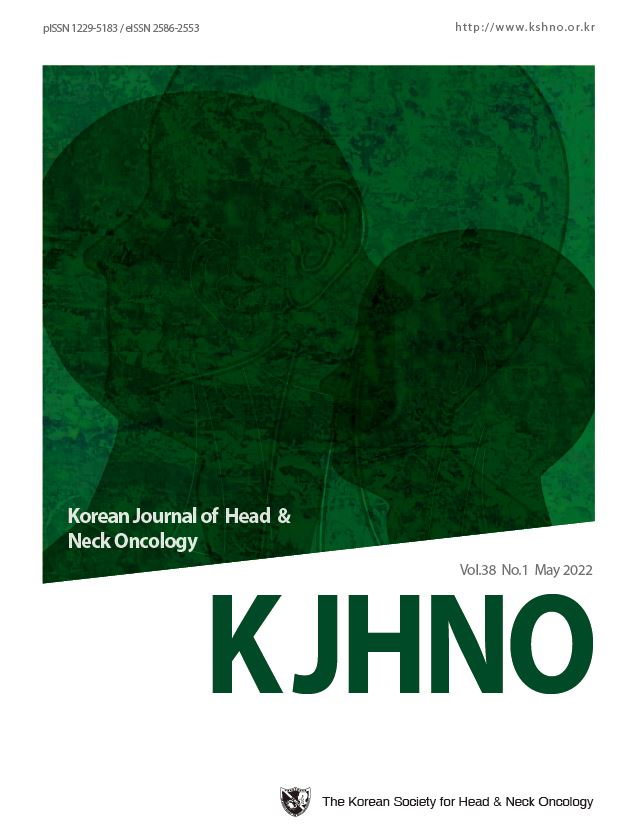
Background/Objectives: This study analyzed the prognostic significance of clinico-pathologic factors including comprehensive nodal factors in parotid gland cancers (PGCs) patients and constructed a survival prediction model for PGCs patients using machine learning techniques. Materials & Methods: A total of 131 PGCs patients were enrolled in the study. Results: There were 19 cases (14.5%) of lymph nodes (LNs) at the lower neck level and 43 cases (32.8%) involved multiple level LNs metastases. There were 2 cases (1.5%) of metastases to the contralateral LNs. Intraparotid LNs metastasis was observed in 6 cases (4.6%) and extranodal extension (ENE) findings were observed in 35 cases (26.7%). Lymphovascular invasion (LVI) and perineural invasion findings were observed in 42 cases (32.1%) and 49 cases (37.4%), respectively. Machine learning prediction models were constructed using clinico-pathologic factors including comprehensive nodal factors and Decision Tree and Stacking model showed the highest accuracy at 74% and 70% for predicting patient’s survival. Conclusion: Lower level LNs metastasis and LNR have important prognostic significance for predicting disease recurrence and survival in PGCs patients. These two factors were used as important features for constructing machine learning prediction model. Our machine learning model could predict PGCs patient’s survival with a considerable level of accuracy.
서론
대상 및 방법
결과
고찰
결론
References
(0)
(0)