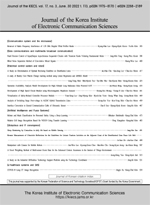
전이 학습을 이용한 VGG19 기반 말라리아셀 이미지 인식
Malaria Cell Image Recognition Based On VGG19 Using Transfer Learning
- 한국전자통신학회
- 한국전자통신학회 논문지
- 제17권 제3호
- : KCI등재
- 2022.06
- 483 - 490 (8 pages)
말라리아는 기생충에 의해 발생하는 질병으로 전 세계에 퍼져있다. 말라리아 셀을 인식하는데 일반적으로 두꺼운 혈흔과 얇은 혈흔 검사 방법이 사용되지만 이러한 방법은 많은 수작업 계산이 필요하여 효율성과 정확성이 매우 낮을 뿐만 아니라 빈민국에는 병리학자가 부족하여 말라리아 치명율이 높다. 본 논문에서는 특징 추출기, 잔류 구조와 완전 연결층으로 구성되고, 전이 학습을 이용한 말라리아셀 이미지를 인식하는 모델을 제안한다. VGG-19 모델의 사전 학습된 파라미터가 사용될 때 일부 컨볼루션층의 파라미터는 고정되고, 모델의 데이터에 맞추기 위하여 미세조정이 사용된다. 그리고 제안된 모델과 비교하기 위하여 잔류 구조가 없는 말라리아셀 인식 모델을 구현한다. 실험 결과 잔류 구조를 사용한 모델이 잔류 구조가 없는 모델에 비하여 성능이 우수 하였으며, 최신 논문과 비교하여 가장 높은 97.33%의 정확도를 보여주었다.
Malaria is a disease caused by a parasite and it is prevalent in all over the world. The usual method used to recognize malaria cells is a thick and thin blood smears examination methods, but this method requires a lot of manual calculation, so the efficiency and accuracy are very low as well as the lack of pathologists in impoverished country has led to high malaria mortality rates. In this paper, a malaria cell image recognition model using transfer learning is proposed, which consists in the feature extractor, the residual structure and the fully connected layers. When the pre-training parameters of the VGG-19 model are imported to the proposed model, the parameters of some convolutional layers model are frozen and the fine-tuning method is used to fit the data for the model. Also we implement another malaria cell recognition model without residual structure to compare with the proposed model. The simulation results shows that the model using the residual structure gets better performance than the other model without residual structure and the proposed model has the best accuracy of 97.33% compared to other recent papers.
Ⅰ. Introduction
Ⅱ. Related work
Ⅲ. Methodology
Ⅳ. Results
Ⅴ. Conclusion
References