통합 측도를 사용한 주성분해석 부공간에서의 k-평균 군집화 방법
K-Means Clustering in the PCA Subspace using an Unified Measure
- 한국전자통신학회
- 한국전자통신학회 논문지
- 제17권 제4호
-
2022.08703 - 708 (6 pages)
-
DOI : 10.13067/JKIECS.2022.17.4.703
- 38
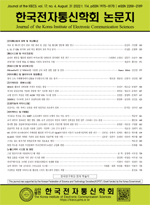
k-평균 군집화는 대표적인 클러스터링 기법이다. 하지만 성능 평가 척도와 최소 개수의 군집을 정하는 방법에 대하여 통합하지 못한 한계가 있다. 본 논문에서는 수치적으로 최소 개수의 군집을 정하는 방법을 도입한다. 설명된 분산을 통합측도로 제시한다. 최소 개수의 군집과 설명된 분산 달성을 동시에 만족하려면 주성분 해석의 부공간에서 k-평균 군집화 방법을 수행해야한다는 것을 제시하고자 한다. 패턴인식과 기계학습에서 왜 주성분 분석과 k-평균 군집화를 순차적으로 수행하는가에 대한 설명을 원론적으로 제시한다.
K-means clustering is a representative clustering technique. However, there is a limitation in not being able to integrate the performance evaluation scale and the method of determining the minimum number of clusters. In this paper, a method for numerically determining the minimum number of clusters is introduced. The explained variance is presented as an integrated measure. We propose that the k-means clustering method should be performed in the subspace of the PCA in order to simultaneously satisfy the minimum number of clusters and the threshold of the explained variance. It aims to present an explanation in principle why principal component analysis and k-means clustering are sequentially performed in pattern recognition and machine learning.
Ⅰ. Introduction
Ⅱ. Explained Variance of PCA
Ⅲ. K-means Clustering Algorithm in the PCA Subspace
Ⅳ. Experimental Results
Ⅴ. Conclusions
References
(0)
(0)