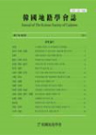
지적재조사사업에서의 무인비행장치와 Mask R-CNN을 이용한 건축물 현황 취득 방안 연구
A Study on the Acquisition of the Status of Buildings Using Unmanned Aerial Vehicle and Mask R-CNN in Cadastral Resurvey Projects
지적재조사사업에서 건축물의 현황 파악은 지적재조사지역 측량조사 시에 수행한다. 이는 지적재조사 측량 시에 건축물 현황 조사를 추가적으로 수행해야 되므로 시간, 비용 손실이 발생하게 된다. 이를 감소하기 위해 무인비행장치 영상과 딥러닝의 Mask R-CNN 알고리즘을 적용하여 건축물에 대한 현황 추출을 진행하였다. 추출된 객체와 지상측량의 경계점 성과를 비교하였다. 비교 결과 RMSE X=0.072m, Y=0.062m로 지적재조사사업에 적용 가능한 값을 도출하였다. 하지만 건축물 현황 추출률이 약 50%이며 추출된 객체의 정확도가 낮은 건축물도 발생하였다. 이는 훈련데이터의 부족 등으로 건축물 명확하게 인식하지 못한 것으로 판단된다. 향후 데이터의 축적과 딥러닝 알고리즘의 발전으로 추출률이 더욱 높아진다면 향후 지적재조사사업 등 지적 분야에 적용이 가능할 것으로 판단된다.
In the cadastral re survey project, the current status of buildings is assessed during the survey of the cadastral re survey area. This results in loss of time and cost because it is necessary to additionally conduct a building status survey during the cadastral re survey. To reduce this, we applied the unmanned aerial vehicle image and the Mask R-CNN algorithm of deep learning to extract the current status of the building. The performance of the extracted object and the boundary point of the ground survey was compared. As a result of comparison, RMSE X = 0.072m, Y = 0.062m, a value applicable to the cadastral resurvey project was derived. However, the extraction rate of the building status was about 50%, and there were also buildings with low accuracy of the extracted objects. It is judged that the building was not clearly recognized due to the lack of training data. If the extraction rate is further increased due to the accumulation of data and the development of deep learning algorithms in the future, it is judged that it can be applied to cadastral fields such as cadastral resurvey project in the future.
1. 서 론
2. 이론적 접근
3. Mask R-CNN를 이용한 건물 경계선 추출
4. 결과분석 및 고찰
5. 결 론