EXoN: EXplainable encoder Network
- 한국자료분석학회
- 한국자료분석학회 학술대회자료집
- 2022년 하계학술대회 발표집
-
2022.0753 - 53 (1 pages)
- 7
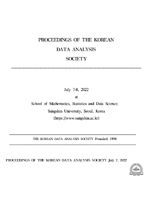
We propose a new semi-supervised learning method of Variational AutoEncoder(VAE) which yields a customized explainable latent space by EXplainable encoder Network(EXoN). Customization means a manual layout design of latent space for specific labeled data. The EXoN provides two useful guides for customization of VAE by a conceptual center and a multi-modality of the latent space. The latent subspace produced by EXoN can be easily investigated by a simple measure. To improve the performance of our VAE in a classification task without the loss of performance as a generative model, we employ a new semi-supervised classification method called `SCI(Soft-label Consistency Interpolation). It is found that both the classification loss and the Kullback-Leibler divergence play a crucial role in constructing explainable latent space and the variability of generated samples from our proposed model depends on a specific subspace, called `activated latent subspace'. Our numerical results with MNIST and CIFAR-10 datasets show that EXoN produces an explainable latent space and reduces the cost of investigating representation patterns on the latent space.
(0)
(0)