ODIM: a method to identify inliers via inlier-memorization effect of deep generative models
- 한국자료분석학회
- 한국자료분석학회 학술대회자료집
- 2022년 하계학술대회 발표집
-
2022.07127 - 129 (3 pages)
- 6
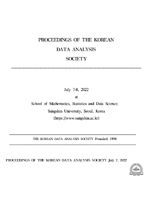
Identifying whether a given sample is an outlier or not is a significant issue in various real-world domains. Many trials have developed outlier detection methods, but they mainly presumed no outliers in the training data set. This study considers a more general situation where training data contains some outliers, and any information about inliers and outliers is not given. We propose a powerful and efficient learning framework to identify inliers in a training data set using deep neural networks. We start with a new observation, called the inlier-memorization effect, that when we train a deep generative model with data contaminated with outliers, the model first memorizes inliers before outliers. Exploiting this finding, we develop a new method called Outlier Detection via the Inlier-Memorization effect(ODIM). The ODIM only requires a few updates; thus, it is fast and efficient. We also provide a data-adaptive strategy to find the optimal number of updates, which makes the ODIM applied to real domains at ease. We empirically demonstrate that our method can refine inliers successfully in both tabular and image data sets.
1. Introduction
References
(0)
(0)