데이터마이닝을 위한 엔트로피 기법과 러프집합이론에 의한 연속 수치 데이터의 속성제거 및 전역 이산화 알고리즘
Removing Attributes and Global Discretization Algorithms of Continuous Numerical Data With Entropy Methods and Rough Set Theory for Data Mining
- 한국의료정보교육협회
- 보건의료생명과학논문지
- Vol.6 No.2
-
2018.12193 - 205 (13 pages)
- 15
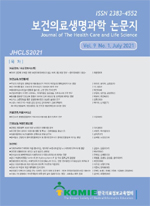
방대한 의료정보와 같은 데이터웨어하우스에는 연속적 수치형 및 이산형 수치형 자료들이 상호 혼재하는 경우가 빈번하다. 일반속성 변수들이 연속적 수치형이거나 이산적으로 혼합되어 있는 경우, 원 데이터세트에 관해 다양한 데이터분석, 데이터마이닝 기법 등의 정보처리를 수행하고자 할 때는 연속적 수치형 데이터를 이산화(discretization)하는 전처리과정이 요구될 경우가 많다. 본 논문에서는 데이터세트의 크기를 축소시키기 위한 목적으로 불필요한 속성을 제거하는 기법과 이와 별개의 알고리즘인 이산화알고리즘 중 전역적 엔트로피기반의 지배속성알고리즘의 특징을 검토하고자 한다. 이와 더불어 서로 별개의 알고리즘이지만 그 이론적 유사성을 통해서 두 목적을 달성하는 통합된 엔트로피 기반의 알고리즘의 가능성을 예시를 통해 논의 하고자 한다.
It is common that continuous and discrete numerical data usually exist in the same dataware house with mixed way, especially like massive medical dataware houses. When conditional attributes consist of mixed data type, we need to necessary to take adequate prerequisite data processing steps. Such a prerequiste steps are discretization steps of continuous numerical data and removing steps of dispensible attribute for reducing the size of dataset. In this thesis, we consider that firstly, the methods of global removing unnecessary attributes and secondly the methods of global discretization algorithm based on entropy. We also consider the possibility of integrating these two kinds of algorithms to develop one new algorithm considering two problems at the same time with representing examples.
1. 서론
2. 러프집합과 정보시스템
3. 엔트로피 기반 전역적 이산화 기법
4. 사례 분석
5. 결론
References
(0)
(0)