Bayesian Inference for continuous time GARCH diffusion limit stochastic volatility models
Bayesian Inference for continuous time GARCH diffusion limit stochastic volatility models
- 한국계량경제학회
- JOURNAL OF ECONOMIC THEORY AND ECONOMETRICS
- Vol.33 No.3
-
2022.0975 - 122 (48 pages)
-
DOI : 10.22812/jetem.2022.33.3.004
- 3
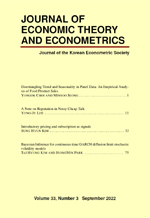
본 연구는 연속시간 GARCH 극한 확률변동성모형에 대한 베이지언 MCMC알고리듬과 알고리듬의 성과에 대한 모의실험 결과, 그리고 실증분석 결과를 제시한다. 본 연구의 알고리듬은 1단계-NR알고리듬(one-step Newton-Raphson algorithm)을 이용한 조건부분산 사후확률밀도함수에 대한정규분포근사를 이용한다. 본 연구의 알고리듬은 GARCH 극한 확률변동성모형 뿐만 아니라 조건부분산의 주변확률밀도함수 또는 주변확률밀도함수의확률커널(probability kernel)을 알 수 있는 연속시간 확률변동성모형 추정에적용될 수 있다. 본 연구의 MCMC알고리듬의 일반성에 대한 예시로 GARCH 극한 확률변동성모형과 함께 Feller제곱근 확률변동성모형에 대한 실증분석결과를 제시한다.
We propose a new Bayesian Markov chain Monte Carlo algorithm for continuous time GARCH diffusion limit stochastic volatility models and demonstrate the performance of our algorithm through simulation experiments and empirical analyses. Our algorithm exploits the normal distribution approximation of the posterior density of conditional variance using the one-step Newton- Raphson algorithm. Our algorithm can be applied not only to the continuous time GARCH diffusion limit stochastic volatility model but also to the continuous time stochastic volatility models of which the marginal probability density functions or the probability kernels of them are known. We present an empirical analysis results of the Feller square-root stochastic volatility model as well as the continuous time GARCH diffusion limit stochastic volatility model as an exhibition of the generality of our MCMC algorithm.
1. 서론
2. GARCH 극한 연속시간 확률변동성모형
3. GARCH 극한 확률변동성모형에 대한 베이지언 추론 알고리듬
4. 모의실험
5. 실증분석
6. 결론
REFERENCES
(0)
(0)