함수형 공간 자료를 위한 추정 및 예측모형과 응용
Estimation and Prediction Model for Functional Spatial Data and Application
- 한국자료분석학회
- Journal of The Korean Data Analysis Society (JKDAS)
- Vol.24 No.5
-
2022.101681 - 1692 (12 pages)
-
DOI : 10.37727/jkdas.2022.24.5.1681
- 45
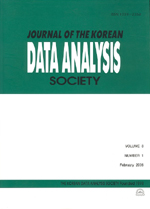
미세먼지는 인체에 유해한 화합물 등으로 구성되어 눈에 보이지 않는 입자로 공기 중에 떠다니며 대기 오염을 유발하거나 인체에 흡입되어 각종 질병을 유발한다. 기상청 개방포털에 의하면 미세먼지는 전국 28개 관측소에서 측정되어 이와 관련한 예보 및 경보 시스템 등에 널리 사용 된다. 본 연구에서는 측정된 미세먼지 자료를 함수형 공간 자료 모형에 의하여 추출된 자료라 가정하고 공간 통계학적 접근 방법과 함수형 자료 분석 방법을 결합하여 고정효과인 전국 평균 함수를 추정하고 예측할 수 있는 기법을 소개하고자 한다. 기존의 연구는 측정된 미세먼지를 설명하는 방법이 경시적 자료 분석법에 집중하였는데 이는 관측소의 위치가 균일하게 분포 되어 있지 않은 현실적 상황과 관측소간 거리의 차이 따라 종속성이 존재할 수 있다는 공간 자료의 특성을 고려하지 않은 접근법이다. 따라서 기존 분석법과 관련한 부분을 함수형 자료로 대체하여 적용하고 크리깅과 같은 공간 통계학적 방법을 적용하여 2021년 한 해 동안 획득된 미세먼지 자료를 분석하고자 한다. 구체적으로 전국 28개 관측소에서 얻어진 자료로부터 전국 미세먼지 평균을 이상치에 덜 민감한 가중평균을 통해 고정효과를 추정하고자 한다. 이를 위하여 경험적 베리오그램 분석을 통하여 공분산 과정을 추론하고 최적의 가중치들을 계산하고자 한다. 또한 어떠한 관측 장소에서도 미세먼지 농도를 예측할 수 있는 크리깅 방법을 소개하고 적용해보고자 한다.
Particulate matter consisting of the chemical compounds harmful to human body and floating in the air as the invisible dust has been affecting on the air pollution or the various disease by inhaling into the human body. According to the Korea Meteorological Administration’s open portal, the particulate matter is measured at 28 sites and is widely used in forecasting and warning systems related to the particulate matter. In this paper, we will introduce an approach that can estimate the fixed effect and predict the future value by incorporating the spatial approach with the functional approach, assuming that the nature of the measurement for the particulate matter is a functional nature. While the existing studies have focused on the longitudinal data approaches, these approaches did not consider the characteristics of the spatial data that can have dependency between the sites and of the irregularity that the sites are not uniformly distributed across the spatial domain. To address this issue, we consider extending the existing approach to the functional data approach and applying the spatial approach such as kriging. Specifically, we consider the weighted mean being insensitive to extreme observations to account for the fixed effect from the real data obtained from 28 sites for 2021 year. To do this, we estimate the covariance process and compute the optimal weight through the empirical variogram analysis. Also, we apply a kriging method that can predict the value associated with the particulate matter at any given observational site.
1. 서론
2. 분석방법
3. 모의실험
4. 자료분석
5. 결론
References
(0)
(0)