딥러닝기반 텍스트 분석을 통한 직업분류시스템 구축에 관한 연구
A study of the establishment of a job classification system with deep learning-based online job posting text analysis
- 한국진로창업경영학회
- 한국진로창업경영학회지
- 제6권 제4호
-
2022.11161 - 179 (19 pages)
-
DOI : 10.48206/kceba.2022.6.4.161
- 174
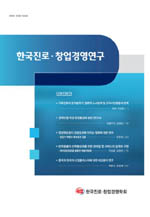
본 연구의 목적은 온라인구인공고 텍스트 데이터를 활용하여 해당 일자리의 직종을 판별하는 분류모델을 생성해 평가하는 것이다. 워크넷 온라인구인공고(OJPs) 텍스트 자료에 딥러닝 기계학습 기법을 적용하여 자동으로 직업을 판별하는 것이다. 텍스트 자동 분류를 위한 기계학습 기법이 규칙기반 모델에서 인공신경망 모델로 전환하는 연구 흐름을 반영하고, 대규모의 온라인구인공고 자료와 텍스트의 문맥적 의미를 잘 다룰 수 있다는 점을 고려하여, 인공신경망의 최신 모델인 Bi-LSTM과 KoBERT 모델을 적용하였다. 1999-2021년 간의 워크넷 구인공고 데이터 800만 개에 모델을 적용한 결과, 0.62-0.82 정도의 매칭 정확도를 달성했다. 특히, 직무 기술(job description)이 특수하고 정확한 전문직에서 높은 정확도를 달성했다.
The purpose of this study is to create a classification model that can identify the type of job by using online job posting text data and evaluate the performance of the model. By applying the latest deep learning machine learning method to Work-Net online job postings(OJPs) text data, it is to automatically determine the occupat ional code of the OJPs. Considering the research trends shifting from a rule-based model to an artificial neural network model. and the merit of handling large-scale online job posting materials and the contextual meaning of text well. the latest models of artificial neural networks. Bi-LSTM and KoBERT models, were applied. As a result of applying the model to 8 million text data of employment insurance Work-Net job posting data from 1999 to 2001 . matching accuracy of 0.62 to 0.82 was achieved. The result is not very high performance. but it is generally judged to be a model that can determine the occupation. In particular. high accuracy was achieved in professions where job descriptions were specific and precise. Although it is not yet perfect for practical use, it is expected that the performance of the automatic occupational classification system will improve in the future when recruitment practices into the job-type labor market change and more precise data pre-processing and model applications are made.
Ⅰ. 서론
Ⅱ. OJPs를 활용한 직업분야 관한 선행연구
Ⅲ. 연구 밥법
Ⅳ. 연구결과
Ⅴ. 결론
참고문헌
(0)
(0)