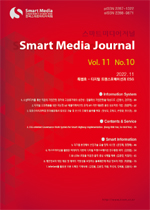
InferSent를 활용한 오픈 도메인 기계독해
Open Domain Machine Reading Comprehension using InferSent
- 한국스마트미디어학회
- 스마트미디어저널
- Vol11, No.10
- : KCI등재후보
- 2022.11
- 89 - 96 (8 pages)
오픈 도메인 기계독해는 질문과 연관된 단락이 존재하지 않아 단락을 검색하는 검색 기능을 추가한 모델이다. 문서 검색은 단어 빈도 기반인 TF-IDF로 많은 연구가 진행됐으나 문서의 양이 많아지면 낮은 성능을 보이는 문제가 있다. 아울러 단락 선별은 단어 기반 임베딩으로 많은 연구가 진행됐으나 문장의 특징을 가지는 단락의 문맥을 정확히 추출하지 못하는 문제가 있다. 그리고 문서 독해는 BERT로 많은 연구가 진행됐으나 방대한 파라미터로 느린 학습 문제를 보였다. 본 논문에서는 언급한 3가지 문제를 해결하기 위해 문서의 길이까지 고려한 BM25를 이용하며 문장 문맥을 얻기 위해 InferSent를 사용하고, 파라미터 수를 줄이기 위해 ALBERT를 이용한 오픈 도메인 기계독해를 제안한다. SQuAD1.1 데이터셋으로 실험을 진행했다. 문서 검색은 BM25의 성능이 TF-IDF보다 3.2% 높았다. 단락 선별은 InferSent가 Transformer보다 0.9% 높았다. 마지막으로 문서 독해에서 단락의 수가 증가하면 ALBERT가 EM에서 0.4%, F1에서 0.2% 더 높았다.
An open domain machine reading comprehension is a model that adds a function to search paragraphs as there are no paragraphs related to a given question. Document searches have an issue of lower performance with a lot of documents despite abundant research with word frequency based TF-IDF. Paragraph selections also have an issue of not extracting paragraph contexts, including sentence characteristics accurately despite a lot of research with word-based embedding. Document reading comprehension has an issue of slow learning due to the growing number of parameters despite a lot of research on BERT. Trying to solve these three issues, this study used BM25 which considered even sentence length and InferSent to get sentence contexts, and proposed an open domain machine reading comprehension with ALBERT to reduce the number of parameters. An experiment was conducted with SQuAD1.1 datasets. BM25 recorded a higher performance of document research than TF-IDF by 3.2%. InferSent showed a higher performance in paragraph selection than Transformer by 0.9%. Finally, as the number of paragraphs increased in document comprehension, ALBERT was 0.4% higher in EM and 0.2% higher in F1.
Ⅰ. 서론
Ⅱ. 관련연구
Ⅲ. 제안 방법
Ⅳ. 실험 및 결과
Ⅴ. 결론
REFERENCES