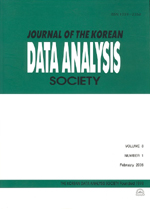
Fundamentals and machine learning in forecast of won-dollar exchange rate
- 한국자료분석학회
- Journal of The Korean Data Analysis Society (JKDAS)
- Vol.24 No.6
- : KCI등재
- 2022.12
- 2099 - 2113 (15 pages)
There are two issues in exchange rate forecast. One is the low explanatory power of economic fundamentals for high frequency exchange rate. The other is the usefulness of machine learning for exchange rate prediction. This paper tries to reconcile those issues by trying to use fundamentals to predict daily exchange rate with machine learning. To match monthly frequency fundamentals with daily exchange rate, linear interpolation is used to transform monthly data to daily. To reduce the noise, daily exchange rates are decomposed to trend and cyclical components using empirical model decomposition (EMD). Regression of trend part of exchange rate on fundamental variables show that the macroeconomic fundamentals have some explanatory power for daily exchange rate. For the forecast, long short-term memory (LSTM) of recurrent neural network (RNN) is applied to trend and cycle part separately. Based on the regression result, fundamental variables are used as input features of LSTM of trend component. Lagged variables are used for LSTM of cyclical part. Forecasts from the two LSTM models are added to get predictors of exchange rate. Results show that trend-cycle LSTM forecasts are better than the traditional random walk predictors.