대응비교를 통한 가우시안 프로세스 회귀모형의 공분산 모수 선택법
The pairwise method for covariance parameter selection in Gaussian process regression model
- 한국자료분석학회
- Journal of The Korean Data Analysis Society (JKDAS)
- Vol.24 No.6
-
2022.122143 - 2155 (13 pages)
-
DOI : 10.37727/jkdas.2022.24.6.2143
- 56
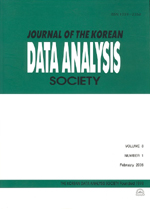
컴퓨터 기술의 발전으로 복잡한 연산의 실험들이 전산 시뮬레이터(simulator)로 수행되고 있다. 시뮬레이터는 계산 비용이 많이 드는 문제가 있어 이를 메타모델(metamodel)을 이용하여 시뮬레이터의 초모수를 최적화 한다. 가우시안 회귀모형(GPRM: Gaussian process regression model)은 메타모델로 많은 연구에 사용되고 있다. GPRM은 회귀계수 와 공분산 모수 로 구성되어 있다. 예측성능이 우수한 GPRM을 위해 선택에 대한 연구는 많이 수행되었으나 선택에 대한 연구는 아직 미흡하다. Welch(1992)는 전진선택법을 이용한 선택 방법을 제안하였다. 본 연구는모든 가능한 대응조합을 이용한 선택 방법을 제안한다. 제안하는 알고리즘은 BIC(Bayes information criterion)와 본페로니 교정(Bonferroni correction)을 이용하여 를 선택한다. 제안 방법은 Welch 알고리즘과 달리 여러 개의 공통된 군이 나타날 수 있으며, 모든 차원의 자료 대신 2 차원의 자료만 고려므로 계산시간이 단축된다. 제안 방법의 성능은 3개의 테스트 함수의 평균제곱근 오차(root mean square error)를 계산하여 비교하였다.
With the development of computer technology, experiments of computer are being performed with a simulator, and the tuning parameters are optimized with metamodel. As a metamodel, the Gaussian process regression model (GPRM) has been used in many studies. GPRM consists of regression coefficient 's and covariance parameter 's. In order to make a better GPRM, many studies have been dealt with the selection of , but only Welch's algorithm (1992) for has been proposed. We propose a selection method using all possible pairwise combinations. The combinations of are classified using the Bayes information criterion(BIC) and Bonferroni correction. Welch's algorithm estimates only a few important ’s individually and estimates the remaining as a single common . In the proposed algorithm, several common ’s may appear depending on the characteristics of , and the calculation time is cheaper because only two-dimensional data are considered. The prediction performance was compared to the Welch's algorithm in the sense of root mean square error (RMSE) through three test functions.
(0)
(0)