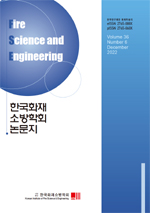
합성곱 신경망 기반 분류 모델의 화재 예측 성능 분석
Analysis of Fire Prediction Performance of Image Classification Models based on Convolutional Neural Network
- 한국화재소방학회
- 한국화재소방학회논문지
- Vol.36 No.6
- : KCI등재
- 2022.12
- 70 - 77 (8 pages)
본 연구에서는 화재 안전 향상을 위한 엣지 컴퓨팅(edge computing) 기반 화재감지시스템에 적용 가능한 합성곱 신경망 기반 이미지 분류 모델들인 MobileNetV2, ResNet101, EfficientNetB0를 이용하여 화재 예측 성능 해석을 수행하였다. 성능평가지표인 정확도, 재현율, 정밀도, F1-score와 혼동 행렬을 이용하여 화재 예측 성능을 비교 분석하였다. 또한 분류 모델의 경량화와 관련한 모델 용량 및 추론시간에 대한 비교 분석을 수행하였다. 비교 분석 결과로서 화재 예측 정확도는 EfficientNetB0 모델이 가장 높았으며 경량성 측면에서는 MobileNetV2가 가장 우수한 것으로 확인하였다. 더하여 화재와 유사한 특징을 갖는 비 화재 이미지인 빛과 연무에 대한 이미지 특성을 추가 학습한 결과, 경량성은 우수하나 예측 성능이 낮은 MobileNetV2의 화재 예측 정확도가 개선되는 것을 확인하였다.
In this study, fire prediction performance was analyzed using convolutional neural network (CNN)-based classification models such as MobileNetV2, ResNet101, and EfficientNetB0 applicable to an edge computing-based fire detection system for improving fire safety. The fire prediction performance was evaluated using the performance evaluation measures including accuracy, recall, precision, F1-score, and the confusion matrix. The model size and inference time were assessed in terms of the light-weight classification model for the practical deployment and use. The analysis results confirmed that the EfficientNetB0 model had the highest fire prediction accuracy, and the MobileNetV2 was the best light-weight classification model. Notably, additionally learning the image features about light and haze images having similar features with those of the fire images improved the fire prediction accuracy of the light-weight MobileNetV2 model.
1. 서론
2. 연구 방법
3. 연구 결과 및 고찰
4. 결론
후기
References