딥러닝을 이용한 직물의 결함 검출에 관한 연구
A Study on the Defect Detection of Fabrics using Deep Learning
- 한국스마트미디어학회
- 스마트미디어저널
- Vol11, No.11
-
2022.1292 - 98 (7 pages)
-
DOI : 10.30693/SMJ.2022.11.11.92
- 85
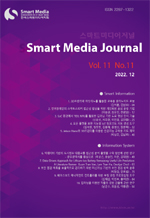
섬유산업에서 생산된 직물의 결함을 식별하는 것은 품질관리를 위한 핵심적인 절차이다. 본 연구는 직물의 이미지를 분석하여 결함을 검출하는 모델을 만들고자 하였다. 연구에 사용된 모델은 딥러닝 기반의 VGGNet과 ResNet이었고, 두 모델의 결함 검출 성능을 비교하여 평가하였다. 정확도는 VGGNet 모델이 0.859, ResNet 모델이 0.893으로 ResNet 모델의 정확도가 더 높은 결과를 보여주었다. 추가적으로 딥러닝 모델이 직물의 이미지 내에서 결함으로 인식한 부분의 위치를 알아보기 위하여 XAI(eXplainable Artificial Intelligence)기법인 Grad-CAM 알고리즘을 사용하여 모델의 관심영역을 도출하였다. 그 결과 딥러닝 모델이 직물의 결함으로 인식한 부분이 육안으로도 실제 결함이 있는 것으로 확인되었다. 본 연구의 결과는 직물의 결함 검출에 있어서 딥러닝 기반의 인공지능을 활용함으로써 섬유의 생산과정에서 발생하는 시간과 비용을 줄일 수 있을 것으로 기대된다.
Identifying defects in textiles is a key procedure for quality control. This study attempted to create a model that detects defects by analyzing the images of the fabrics. The models used in the study were deep learning-based VGGNet and ResNet, and the defect detection performance of the two models was compared and evaluated. The accuracy of the VGGNet and the ResNet model was 0.859 and 0.893, respectively, which showed the higher accuracy of the ResNet. In addition, the region of attention of the model was derived by using the Grad-CAM algorithm, an eXplainable Artificial Intelligence (XAI) technique, to find out the location of the region that the deep learning model recognized as a defect in the fabric image. As a result, it was confirmed that the region recognized by the deep learning model as a defect in the fabric was actually defective even with the naked eyes. The results of this study are expected to reduce the time and cost incurred in the fabric production process by utilizing deep learning-based artificial intelligence in the defect detection of the textile industry.
Ⅰ. 서론
Ⅱ. 관련 연구
Ⅲ. 연구 방법론
Ⅳ. 연구 설계
Ⅴ. 연구 결과
Ⅵ. 결론
REFERENCES
(0)
(0)