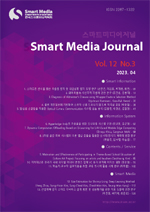
Diagnosis of Alzheimer’s Disease using Wrapper Feature Selection Method
Diagnosis of Alzheimer’s Disease using Wrapper Feature Selection Method
- 한국스마트미디어학회
- 스마트미디어저널
- Vol12, No.3
- : KCI등재후보
- 2023.04
- 30 - 37 (8 pages)
Alzheimer’s disease (AD) symptoms are being treated by early diagnosis, where we can only slow the symptoms and research is still undergoing. In consideration, using T1-weighted images several classification models are proposed in Machine learning to identify AD. In this paper, we consider the improvised feature selection, to reduce the complexity by using wrapping techniques and Restricted Boltzmann Machine (RBM). This present work used the subcortical and cortical features of 278 subjects from the ADNI dataset to identify AD and sMRI. Multi-class classification is used for the experiment i.e., AD, EMCI, LMCI, HC. The proposed feature selection consists of Forward feature selection, Backward feature selection, and Combined PCA & RBM. Forward and backward feature selection methods use an iterative method starting being no features in the forward feature selection and backward feature selection with all features included in the technique. PCA is used to reduce the dimensions and RBM is used to select the best feature without interpreting the features. We have compared the three models with PCA to analysis. The following experiment shows that combined PCA &RBM, and backward feature selection give the best accuracy with respective classification model RF i.e., 88.65, 88.56% respectively.
Ⅰ. INTRODUCTION
Ⅱ. METHODOLOGY
Ⅲ. EXPERIMENT RESULT & DISCUSSION
Ⅳ. CONCLUSION